Synthetic Data and Health Equity
Synthetic Data and Health Equity
ABSTRACT
The lack of diverse health data has long been a worry for physicians, public health workers, healthcare researchers, and others who are concerned about health inequities in the United States. More data, they argue, that reflects the health issues of a larger number of communities is vital to providing healthcare that heals and fosters greater wellness among a broad population. However, robust, diverse health datasets are difficult to obtain due to historic, systemic, and structural barriers to quality data collection that represents and serves the interests of racialized and marginalized populations. Generative artificial intelligence, according to a growing number of healthcare analysts and researchers, might offer a long-sought solution to the scarcity of diverse health data through synthetic data, artificially generated data produced by digital technologies rather than produced by real-world events. These emerging discussions about how generative AI models may be able to fill the gaps in health data with synthetic data also raise important questions about data ethics and the development of appropriate tools for the efficacy of computer-generated health data. This field review highlights key ideas about diversity in health data and interventions to address this issue. Specifically, it explores discussions about generative artificial intelligence and synthetic data in a historical context, with an eye toward the ethical and equity implications of expanding the use of synthetic data to artificially diversify health data. The review concludes by considering the implications of synthetic data in developing processes for creating transformative health outcomes that advance health equity.
Keywords: Algorithmic Bias, Artificial Intelligence, Generative AI, Health Data Poverty, Health Equity, Health Inequality, Synthetic Data
Introduction
A quick Google search for the phrase “AI and healthcare” will yield numerous articles predicting that artificial intelligence (AI) is the “next frontier” of health and well-being. The potential capabilities of AI are certainly seductive and inspire optimism. For example, a recent study reveals that AI can sort through unstructured (textual) data in electronic health records to identify patients with critical social needs that make them more vulnerable to disease and illness (Guevara et al. 2024). However, the researchers indicate that their findings were limited by training and validation datasets that represented a predominantly White population treated at hospitals in Boston, MA. Thus, despite the promise of AI, the lack of diverse data to train AI applications remains a well-documented problem (Anmol et al. 2023). To solve the data sourcing issues, the machine-learning community suggests using AI to generate high-quality text, images, and other content based on algorithms trained on existing data. In short, they argue that AI can create synthetic data, data generated by algorithms, to fill in the gaps where diverse data is hard to obtain or none exists.
On the surface, it seems that AI could generate richer and more nuanced data than real-world data to reflect larger numbers of people, particularly if that data can be used to create health equity. However, great caution is necessary. A careful examination of the factors responsible for the lack of diverse data as well as the methods and metrics for generating synthetic data to fill the void is in order. Inattention to these factors sets synthetic data up to exacerbate, rather than productively address, health inequity.
A Historical Review of Health Data and Race
Behind every set of data lies a story that illustrates a dataset’s limitations in offering an objective truth. The history of quantitative data on health outcomes provides insight into how it became the primary evidence to document and validate racial health disparities. This history offers a better understanding of the politics behind contemporary calls for more data.
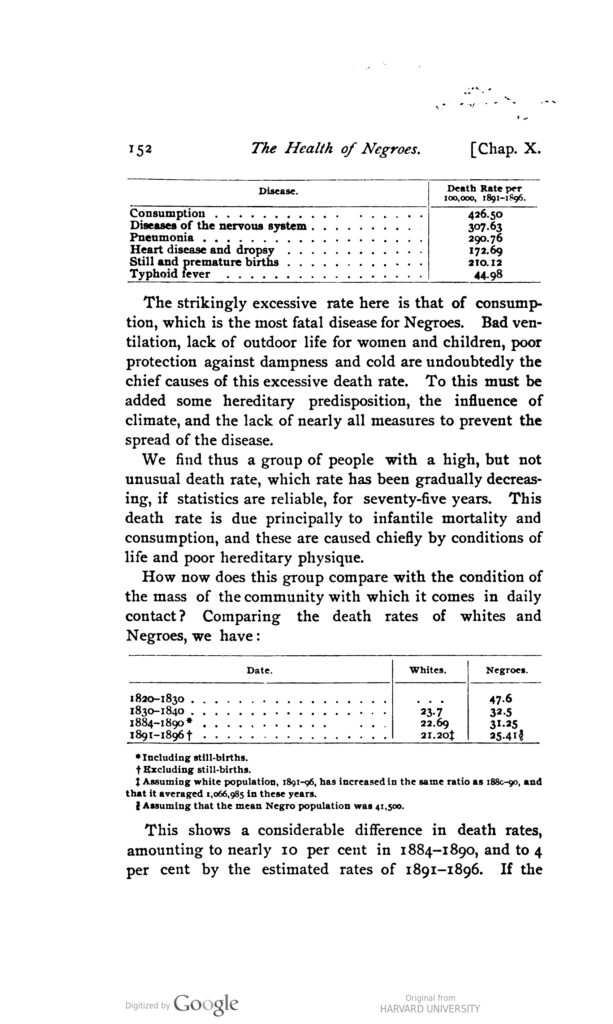
Some of the most important historical uses of data to provide empirical knowledge about racial inequity in health in the United States can be traced to two Black researchers: James McCune Smith, a physician, and W. E. B. Du Bois, a sociologist and activist. As the first professionally trained Black physician in the United States, McCune argued in 1850 that the disparate rates of disease and illness among enslaved Black people were due to their poor living conditions and not to innate racial differences, the common medical viewpoint at the time (Morgan 2003). Similarly, Du Bois, in his 1899 study, The Philadelphia Negro, demonstrated that, contrary to contemporary medical and scientific thinking, differences in health outcomes were not attributed to biological differences, but to environmental and social inequity (Du Bois [1899] 2023). Du Bois was one of the first researchers to use data to argue that factors such as unemployment, poor housing, and inadequate food—what we in the twenty-first century would call social determinants of health—accounted for why Black people in Philadelphia tended to get sick and die earlier than their White counterparts (Williams and Sternthal 2010). Both McCune and Du Bois’s arguments countered the late nineteenth-century ascent of eugenics and scientific racism, a set of beliefs and practices that aim to prove that the genetic makeup of non-White people was inferior. Despite pioneering data-driven approaches to understanding health differentials, the White science and medical community largely failed to attribute racial disparities to social conditions over much of the early twentieth century. Instead, they collected data, for example on tuberculosis, to study the epidemiological structure of the disease to make arguments for racial pathologies—the belief that certain racial groups, such as Black people, were predisposed to specific diseases (Roberts 2009).
By the 1930s, a small but growing number of government and private agencies began to heed Black physicians and scholars of Black life who continued to call attention to socioeconomic data as the root of health disparities. This, along with advances in medicine and therapeutics, meant that “proposing the importance of racial predisposition over the sociomedical aspects of the disease became harder to defend” (Roberts 2009, 78). However, it would take approximately another 50 years for the federal government to adopt data and research methods to study health disparities as socially situated as opposed to racially determined.
The Heckler Report made it clear that disparities in the burden of illness and death experienced by minoritized communities were not solely related to physiological factors, noting that “the factors responsible for the health disparity are complex and defy simplistic solutions.”
In 1985, Margaret Heckler, the health and human services secretary for the Reagan administration, built on the work of the Association of Minority Health Professions, which had documented racial life expectancy disparities in a 1983 study (Hangt, Fishman, and Evans 1983), to produce the first coordinated federal effort to collect health disparity data on a much broader scale. The Report of the Secretary’s Task Force on Black and Minority Health, also known as the Heckler Report, a landmark publication by the Department of Health and Human Services (DHHS), attempted to examine the health issues impacting racially marginalized communities in the late twentieth century. The Heckler Report made it clear that disparities in the burden of illness and death experienced by minoritized communities were not solely related to physiological factors, noting that “the factors responsible for the health disparity are complex and defy simplistic solutions” (Task Force on Black and Minority Health 1985, 7). This statement reinforced the work of early Black health researchers that pointed to environmental causes for health disparities and dramatically departed from centuries of scientific racism. One of the most cited findings from the Heckler Report concluded that health disparities accounted for approximately 60,000 excess deaths of Black people between 1979 and 1981 and that six causes of death (heart disease and stroke; homicide and accidents; cancer; infant mortality; cirrhosis; diabetes) accounted for more than 80 percent of mortality among Black people and racially marginalized communities (Task Force on Black and Minority Health 1985). The Heckler Report spawned a series of conferences and symposiums that sought to respond to the report’s findings, and it inspired the development of the Office of Minority Health in 1986 under the DHHS.
Despite the extensive attention paid to disproportionate Black mortality rates, the call and stated need for more public health data on people of color emerged as an equally impactful conclusion of the Heckler Report. The task force stated:
Reliable data are central to measuring progress in public health and are the key to assessing the current health status of the Nation and measuring health status trends; recognizing both sources of and solutions to the problems; identifying health disparities between segments of the population; and targeting efforts directly to specific needs. (Task Force on Black and Minority Health 1985, 31)
Specifically, the task force revealed that national health data was limited or completely lacking for Hispanic, Asian American, and American Indian/Alaska Native populations. The Heckler Report made the lack of data a central focus by noting how it was especially challenging to obtain health information on racial communities because they are “growing rapidly, changing rapidly, highly mobile, and therefore, difficult to track yet have greater health problems than nonminorities” (Task Force on Black and Minority Health 1985, 31). The task force also identified inconsistencies in data collection practices of ethnic and racial identifiers across different US public health systems.
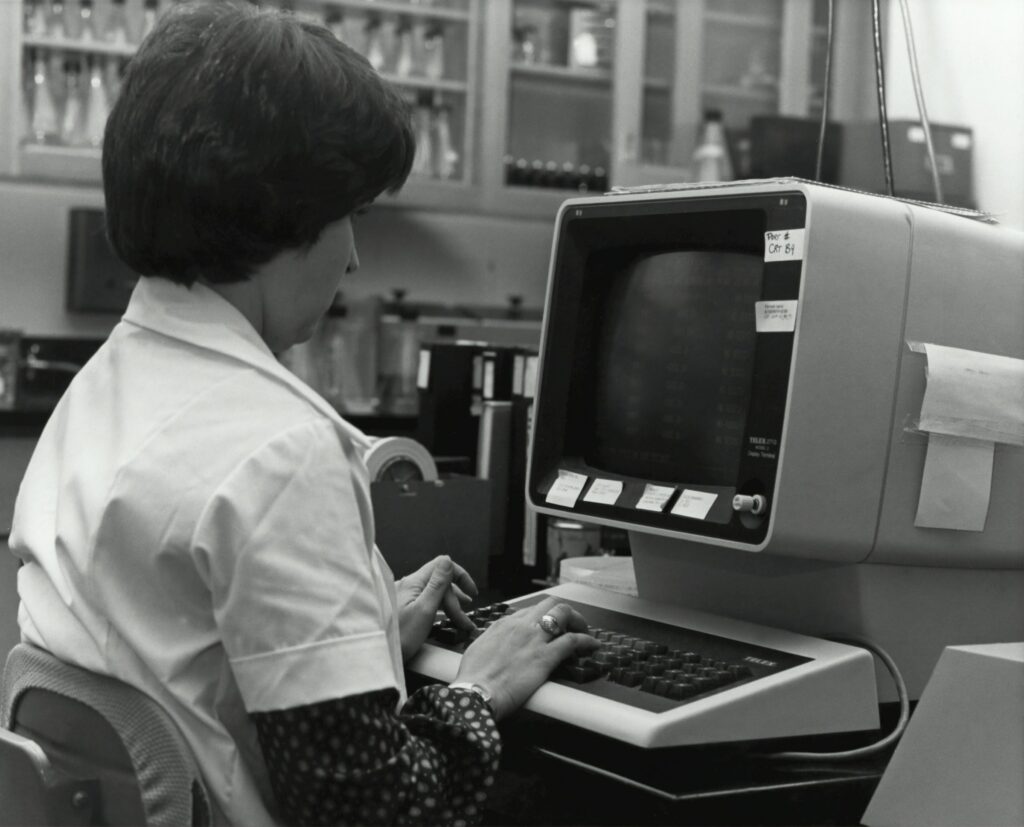
Although the Heckler Report effectively used data to debunk the idea that racially minoritized communities were biologically inferior, hence more likely to get sick and die than White people, it unwittingly created another naturalizing discourse: Public health data on Black people and other communities were inherently difficult to collect. This premise shapes the contemporary discussion on data and health equity that, first, argues for the necessity of racially representative data and, second, that collecting data on communities of color is a problem that must be addressed to combat racially disparate health outcomes Thus, the discourse around the drive for race-based data to prove the existence of health disparities sets up a corollary discussion of the “hard-to-reach population,” an ethnic or racial community that researchers describe as difficult to reach or involve in public health programming (Shaghaghi, Bhopal, and Sheikh 2011). It’s this relationship between, what Rinaldo Walcott (2020) describes as “the positivism of the call for raced-based data,” and the challenges of collecting data from racially minoritized groups that has given birth to the concept of health data poverty.
Health Data Poverty
Since the publication of the Heckler Report, the call for more data to advance health equity has grown in frequency and tenor. In fact, the stakes are even higher for quality data in the twenty-first century where health information is completely digitized and artificial intelligence is being used in medicine. Health professionals, including physicians and public health experts, continue to attribute poor health outcomes, in part, to what is now known as “health data poverty”—the underrepresentation or insufficient inclusion of diverse populations in the data used for healthcare research, analysis, and decision-making (Ibrahim et al. 2021). Today, health data—defined as “any of the clinical, biochemical, radiological, molecular, and pathological information pertaining to a patient that is captured by healthcare professionals and, increasingly, digitally recorded and stored in electronic patient health records” (Ibrahim et al. 2021, e260)—is used in a variety of capacities to improve and maintain the health and wellness of individuals and communities within and outside of healthcare settings.
The value and critical necessity of health data have grown in a digital healthcare world driven by artificial intelligence.
Healthcare professionals rely on data to diagnose and treat patients as well as to develop and enhance healthcare services. Just as important, researchers who are employed in the health industry, higher education, and government collect and analyze data as an element of research methodologies to evaluate current health trends, identify risks to public health, and collaborate with medical health professionals to create health equity for diverse populations. Theodora Kokosi and Katie Harron (2022, 1) state, “Use of information from clinical trials and electronic health records of large populations has the potential to benefit medical and healthcare research and makes seeking new approaches to data access imperative.” In addition, everyday people place value on health data across different spectrums and understand it as essential to health. Individuals use personal devices to track their health data and access their personal health records to maintain and advance their well-being. Thus, the value and critical necessity of health data have grown in a digital healthcare world driven by artificial intelligence. According to DHHS, AI needs “high-quality, clean, and accurate data to fuel the development of algorithms” (CODE 2019, 5). Like the Heckler Report, a common discursive framework for discussing the need for health data in the twenty-first century is to provide evidence for health inequities or what has traditionally been defined as health disparities, which the CDC (2023) defines as “preventable differences in the burden of disease, injury, violence, or in opportunities to achieve optimal health experienced by socially disadvantaged populations.”
While the Heckler Report broadly described the challenges of collecting ethnic and racial health data, contemporary explanations for health data poverty are much more nuanced, and they generally focus on poor data collection practices relative to communities of color. Historical biases and systemic inequities around race, health, and data, as described above, have influenced contemporary health data collection practices, resulting in the underrepresentation of Black, Indigenous, Asian Americans, and Hispanic people, marginalized rural White communities, and other underserved populations such as disabled people. Contemporary clinical data collection methods, for example, may inadvertently exclude or underrepresent certain racial, ethnic, gender, socioeconomic, and age groups (Carter-Edwards et al. 2023). Other, studies or surveys may primarily recruit participants from specific geographic areas, institutions, or populations, leading to a skewed representation of the overall population (Khan et al. 2020).
Privacy and consent requirements can also make it challenging to create diverse datasets for clinical trials and electronic health records. Stricter regulations, ethical considerations, and concerns about data protection may limit the sharing or use of data from underrepresented communities. Along with this, disparities in access to digital technologies and healthcare infrastructure can result in limited participation of certain groups in digital health initiatives or data collection efforts. This can contribute to a lack of representation of those populations in health datasets.
Like health researchers of the past, health professionals in the twenty-first century have raised the alarm on how health data poverty poses great harm, particularly to minoritized communities, and can lead to biased or incomplete findings, which hinder health equity. However, Jean-Baptiste Cazier et al. (2020, 2) go further, noting that “limited return in actionable health improvement, combined with data becoming an important tradable commodity has led to an increased mistrust of effort in health data collection: Populations have begun to feel like a commodity rather than a beneficiary.”
Still, concerns about health data poverty in digital healthcare largely operate off a similar logic found in the Heckler Report. Machine learning engineers require large amounts of data to develop healthcare algorithms—“a computation, often based on statistical or mathematical models, that helps medical practitioners make diagnoses and decisions for treatments and artificial intelligence (AI), to diagnose patient illnesses, suggest treatments, predict health risks, and more” (Colón-Rodríguez 2023). The damage, then, that data poverty portends is compounded in a healthcare world dependent on data-driven decisions and health information that is created and delivered through digital technology.
The consequences of the dearth of diversity in health data and digital health … can lead to biased or incomplete insights into health conditions, treatment responses, and health disparities among different population groups.
When machine learning engineers use data that does not reflect a cross-section of society, they run the risk of creating faulty algorithms and AI bias that range from misdiagnosing health issues to creating digital health technologies that are misaligned with patients’ health needs. For example, “many algorithms are trained or tested on the International Skin Imaging Collaboration (ISIC) dataset…but lack images of inflammatory and uncommon diseases, or images across diverse skin tones” (Daneshjou et al. 2022, 1). The lack of diversity in health data is not isolated to race. Research has demonstrated that some prediction models for cardiovascular disease are trained on predominantly White male data and may be faulty in diagnosing women’s vulnerability to heart attacks (Norori et al. 2021). Therefore, the consequences of the dearth of diversity in health data and digital health can be significant. It can lead to biased or incomplete insights into health conditions, treatment responses, and health disparities among different population groups. This can result in less effective healthcare interventions, produce inaccurate risk assessments, and engender inequitable healthcare practices.
Though the rationale for finding ways to end health data poverty echoes that of the late twentieth century, the sheer quantity of data necessary to develop AI has shifted some of the underlying principles of acquiring more diverse health data (Sehgal 2023). Rather than data sourcing to achieve health equity, health data for AI may “provide grist for the mill for computer scientists and researchers interested in developing AI technology” (London 2022, 2). In other words, the desire for data for AI medicine may be less about solving health disparities on their own and more about developing efficient algorithms. In scenarios such as this, data has the potential to become a problem and not a path to a well-needed medical intervention that has the potential to create health equity.
Synthetic Health Data
Machine learning engineers’ recent advocacy for synthetic data as a solution to the data problem in AI health technology is a significant turn in the overall discussion about health equity. Synthetic data is artificially generated data that imitates real-world data but does not contain any personally identifiable information (PII) or sensitive information (Martineau and Feris 2023). Computer scientists and researchers use algorithms, statistical models, or other computational techniques to simulate data that closely resembles the characteristics, patterns, and distributions of real data. The purpose of generating synthetic data is to provide a substitute for real data when it is difficult to obtain or privacy, security, or legal concerns restrict its use. Synthetic data allows machine learning engineers to perform various tasks, such as testing and developing algorithms, conducting simulations, training machine learning models, and performing statistical analyses. A key aspect of synthetic data is that it can include statistical properties such as distributions of continuous data and correlations between variables that mirror the original data.
The use of synthetic data in healthcare research and education is far from unique nor is it new. Clinical educators regularly use simulated cases and patients to teach medical students about the progression of disease and different forms of treatment (Metcalf, Rossie, and Workman 2020). However, computer science has made creating synthetic health data much easier. Diverse scenarios, populations, or situations that may not be readily available with real-world data can be made available through technology. These custom computer-generated datasets that encompass a wide range of variables, distributions, and relationships can aid in diagnosis, treatment, and insight into health and wellness. Most recently, Jason Walonoski et al. (2020) created 124,150 synthetic patients to develop a model of the Covid-19 disease progression and treatment for Synthea, an open-source, synthetic patient generator program led by the Office of the National Coordinator for Health Information Technology (ONC) and developed by the MITRE Corporation that ran between 2019 and March 2022. Synthea created synthetic patients with a combination of algorithms and rules that produced SyntheticMass, a dataset that emulated the medical and demographic features of healthcare data of Massachusetts residents.
The development of generative AI, deep-learning models that can take raw data and “learn” to generate statistically probable outputs when prompted, has resulted in a full-throated promotion from many in the machine learning community for replacing real-world data with synthetic data. Much of the technology responsible for the ability to create synthetic data and that is driving AI chatbots, such as ChatGPT and Gemini by Google, can be traced to Ian Goodfellow. In 2014, Goodfellow, then a PhD student at the Université de Montréal, led a group of computer scientists in developing an unsupervised learning framework, algorithms that are trained on unlabeled training data to learn, predict, or reproduce new data. This came to be known as “Generative Adversarial Networks” or “GANs” to describe two competing AI models (the generator and the discriminator) that produced synthetic images (Goodfellow et al. 2014; 2020).
The development of GANs accelerated the sophistication of machine learning, because it does not rely on human supervision of the data model to learn how to recognize or reproduce data. In the case of synthetic data, it attempts to reproduce a copy of it. Machine learning occurs as a generator learns what is real from a discriminator’s classification of real and fake information that is inputted into a computer system. One of the significant consequences of GANs is that over time the generator not only gets better at detecting counterfeit outputs but also at producing things that mimic the “real.” This is both a benefit and a drawback. AI-generated deepfakes are a known and growing problem that policymakers and tech developers are scrambling to address.
Some of the optimism surrounding synthetic data’s potential for diversifying health datasets has been dampened by computer scientists who claim that GANs and other AI models are not ready for primetime, noting that they might cause more harm than good at the present time.
Despite the concerns about GANs and other generative models, it has made synthetic data the next big thing in the tech world. Alexander Linden at Gartner, Inc., an American technological research firm, predicts that by 2030, the majority of data used for AI and analytics projects overall will be synthetic (Goasduff 2022). In 2020, The Massachusetts Institute of Technology (MIT) announced the release of the “Synthetic Data Vault,” “a one-stop shop where users can get as much data as they need for projects” (Laboratory for Information and Decision Systems 2020). When it comes to healthcare, Mauro Giuffrè and Dennis Shung (2023, 3) state, “The incorporation of synthetic data in healthcare has been lauded for its potential to circumvent the challenges surrounding data scarcity.” Yet some of the optimism surrounding synthetic data’s potential for diversifying health datasets has been dampened by computer scientists who claim that GANs and other AI models are not ready for primetime, noting that they might cause more harm than good at the present time. Indeed, generative AI models may work too efficiently in creating data that too closely matches real-world data. Chief Technology Officer David Talby (2023) at John Snow Labs, an AI company that works with healthcare and life science organizations to implement AI technologies across their processes, states, “Synthetic data is often ‘too clean,’ as it lacks the inherent noise and variability present in real-world patient data.” Therefore, as Talby points out, synthetic data captures the statistical properties of the original data but fails to include critical elements to understanding the nuances of health conditions, such as lifestyle and social determinants of health. This distorts any conclusions that might be derived from the data and, in one instance he cites, clean data skewed the prognosis of Parkinsonian gaits based on synthetically generated images.
Another obvious but key disadvantage of synthetic data is that it is heavily dependent on the quality of the real data. If the real dataset contains inaccuracies or biases, the synthetic dataset will likely contain the same problem. Talby (2023) writes, “Generative algorithms are only as good as the data they are trained on, and any bias in the source data will be reflected in the generated synthetic data.” Effective AI models require quality and diverse datasets for machine learning. In this regard, “multi-institutional datasets that capture a larger diversity of clinical phenotypes and outcomes” are integral to training generative AI models (Chen et al. 2021, 495). However, Giuffrè and Shung (2023, 3) also warn that synthetic data may “unintentionally disclose identifiable details about individuals or lead to re-identification, violating privacy, and data protection principles.”
One of the most important and concerning issues about synthetic health data is that there is very little oversight and standardization over the AI models creating it. Ian Goodfellow et al. (2020, 143) note, “Evaluating the performance of generative AI models including GANs is a difficult research area in its own right.” Ghadeer Ghosheh, Jin Li, and Tingtin Zhu (2022, 17) write, “Synthetic data generated for data augmentation in machine learning tasks should be evaluated differently than generating research purposes or imputing missing values and estimating counterfactuals, which might go beyond the predictive utility of the data.” In other words, some researchers express concern about the use of machine learning on synthetic data that may produce algorithms that involve clinical care and treatment of patients.
Although health data poverty is a pervasive problem that poses challenges to health equity, the questions that critics pose about AI dispute the argument that synthetic data is the solution to the lack of diversity in data for AI in medicine and healthcare. Ironically, some of the criticism that has given some computer scientists a moment for pause in moving forward with synthetic data in healthcare has pushed machine learning engineers to advance a discourse about efficient algorithms as opposed to one that is centered on ethics and health equity.
The Ethics of Synthetic Health Data
Despite the early ethical and technical concerns raised by critics, advocates continue to argue that “in medicine and healthcare accurate synthetic data can be used to increase diversity in datasets and to increase the robustness and adaptability of AI models” (Chen et al. 2021, 493). The emphasis on accuracy means that machine learning engineers have started to establish metrics for measuring the “fairness” of synthetic data based on whether it is representative of diverse populations. Much of these evaluative metrics are based on “fairness models” established by the tech community to address “algorithmic bias” that activists and scholars such as Ruha Benjamin, Joy Buolamwini, Timnit Gebru, Safiya Umoja Noble, Cathy O’Neil, Rumman Chowdhury, and Seeta Peña Gangadharan have identified in AI (O’Neil 2023).
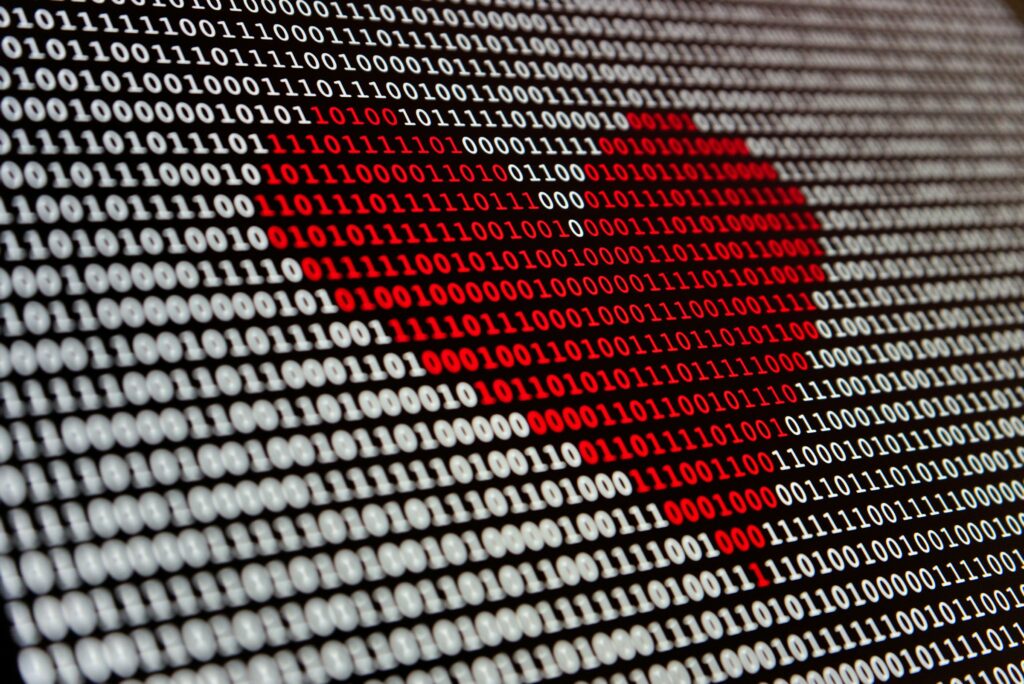
Fairness and efforts to correct algorithmic bias in automated decision processes, according to the tech industry, have the potential to correct bias in machine learning. Raffale Marchesi et al. (2022) have created specific computational techniques to mitigate algorithmic bias stemming from health data poverty, where minoritized patients are underrepresented in training datasets for machine learning. Based on mathematical calculations that measure covariate-level fairness—different factors such as race, gender, and socioeconomic status that might affect the outcome—in synthetically generated healthcare data, Karan Bhanot et al. argue that they have created an “equity metric” to create fair “synthetic versions of healthcare data [that] faithfully represent diverse minority subgroups” (2021, 2) as well as “capture the intrinsic trends and patterns in the real data, while simultaneously preserving the privacy of subjects in all subgroups” (2021, 3). Other researchers have proposed a pipeline method that removes bias-inducing samples from the training dataset used in generative AI models to increase the likelihood that synthetic data has little or none of the biased characteristics that might be found in real-world data (Chaudhari et al. 2022). The number of efforts to establish different metrics for generating fair synthetic data has sparked discussion among the machine learning community about which approach is more productive. However, no real efforts exist, yet, to establish standards for creating and validating the quality of synthetic health data when it comes to addressing health disparities and, more broadly, healthcare overall (Gonzales, Guruswamy, and Smith 2023).
In sharp contrast to historical discussions about the need for better health data to address health inequities, discourse about health data in the context of AI is more focused on computational methods for making efficient algorithms to assemble and process data. While this may have downstream benefits that tackle health disparities—though no clear evidence suggests that it does—it has the unintended, but negative consequence of eclipsing endeavors to develop better data collection practices that have the potential of improving patients’ relationships with public health workers, physicians, and healthcare researchers. For instance, the Heckler Report outlined a list of strategies that should be taken to improve data collection for racially underrepresented communities, including requiring all DHHS agencies to collect data on race and ethnicity, as outlined by the Office of Management and Budget, and “strengthen and expand cooperative efforts to train personnel to complete vital statistic records accurately” (Task Force on Black and Minority Health 1985, 35). The All of Us Research Program, created by the National Institutes of Health under the Obama administration in 2015, is one of many strategies that built on the Heckler Report’s efforts to develop better health-data collection practices (“Improving Data Collection” 2018). In seeking to address some of the systemic challenges to acquiring diverse health data, to date, close to half a million people have completed surveys, agreed to share their electronic health records, provided physical measurements, and donated at least one biospecimen. Painstakingly, in its goal to gather health data from over a million people, the All of Us ResearchProgram is guided by a core value that “transparency earns trust.” In short, the Heckler Report and programs like All of Us recognize that ending health data poverty starts with people’s relationship to data.
Machine learning engineers’ determination to create synthetic data that offers all the benefits of real-world data without the messy challenges that naturally emerge with human-centered data collection processes may leapfrog over the necessary, albeit tough, steps that result in more transformative change and potential for improved health outcomes.
In a machine learning world where synthetic health data is privileged over hard-earned data that is the dint of informed consent, time, and trust, computational processes function as a quick and relatively easier data collection praxis to fuel AI innovation. More traditional health data collection instruments, such as questionnaires, surveys, focus groups, patient self-reported data, and medical records, require time and people. Historically, these data collection practices have challenged efforts to obtain the necessary data to advance health equity. At the same time, they have often created space for deliberate and methodical processes that seek to prioritize people’s health needs and more trustful relationships between the healthcare system and patients. This begs the question of whether generative AI models will be aligned with real-world data that reflect health priorities to train algorithms rather than data that is simply easier to use for machine learning. Consequently, machine learning engineers’ determination to create synthetic data that offers all the benefits of real-world data without the messy challenges that naturally emerge with human-centered data collection processes may leapfrog over the necessary, albeit tough, steps that result in more transformative change and potential for improved health outcomes. Alex John London notes that “the problems that stakeholders are often trying to overcome through the use of AI or other computational models are the result of problems that operate at a larger social level” (Bahls 2023). Generative AI models, in this sense, that yield synthetic health data may increase the amount of tabular information available to researchers and clinicians. However, whether these models will create useful data that result in transformative health outcomes that move the needle toward health equity remains to be seen.
Conclusion
Health data poverty, at least for the time being, continues to be a significant problem in addressing health disparities. Many people in the machine learning community have expressed great optimism about generative AI models and other technologies’ capacity to create synthetic health data to fill the gap left by scarce data sources. Because discussions around the potential of synthetic data to address issues of bias, privacy, and health data poverty are so new, many researchers, healthcare professionals, and technology scholars are unfamiliar with the topic. Therefore, the ethical issues that surround the generation of synthetic health data have yet to be fully explored, and it is unclear who will be responsible for addressing them on a systemic level at this juncture. Technology writer David Gilad Maayan (2022) predicts that “in many cases, it will be an ML engineer who will make the call—do we need real data, or can we settle for synthetic data, for a given problem.” However, this does not have to be the case. Recognizing the critical need for more diverse health data, healthcare professionals, researchers, health informaticists, and public health workers along with communities of color are working to develop a variety of tactics to augment and increase health data resources. Central to these approaches are building trustworthy relationships with Black and Brown communities. Computer scientists and machine learning engineers must reach out to these individuals and groups as well as to scholars and activists working to solve algorithmic bias to think through the implications of using synthetic data in healthcare. Just technology and health equity require nothing less.
Recommended Readings
Benjamins, Maureen R., and Fernando G. De Maio, eds. 2021. Unequal Cities: Structural Racism and the Death Gap in America’s Largest Cities. Health Equity in America. Baltimore, MD: Johns Hopkins University Press.
Matthew, Dayna Bowen. 2018. Just Medicine: A Cure for Racial Inequality in American Health Care. New York: New York University Press.
Panch, Trishan, Heather Mattie, and Leo Anthony Celi. 2019. “The ‘Inconvenient Truth’ about AI in Healthcare.” npj Digital Medicine 2. https://doi.org/10.1038/s41746-019-0155-4.
Pollock, Anne. 2021. Sickening: Anti-Black Racism and Health Disparities in the United States. Minneapolis: University of Minnesota Press.
The White House Office of Science and Technology Policy (OSTP). n.d. “Blueprint for an AI Bill of Rights.” The White House. https://www.whitehouse.gov/ostp/ai-bill-of-rights/.
References
Anmol, Arora, Joseph E. Alderman, Joanne Palmer, Shaswath Ganapathi, Elinor Laws, Melissa McCradden, Lauren Oakden-Rayner, et al. 2023. “The Value of Standards for Health Datasets in Artificial Intelligence-based Applications.” Nature Medicine 29: 2929–2938. https://doi.org/10.1038/s41591-023-02608-w.
Bahls, Christine. 2023. “Data Equity: Representing Underrepresented Populations.” Applied Clinical Trials, March 6, 2023. https://www.appliedclinicaltrialsonline.com/view/data-equity-representing-underrepresented-populations.
Bhanot, Karan, Miao Qi, John S. Erickson, Isabelle Guyon, and Kristin P. Bennett. 2021. “The Problem of Fairness in Synthetic Healthcare Data.” Entropy (Basel) 23 (9): 1165. https://doi.org/10.3390/e23091165.
Carter-Edwards, Lori, Bertha Hidalgo, Freda Lewis-Hall, Tung Nguyen, and Joni Rutter. 2023. “Diversity, Equity, Inclusion, and Access Are Necessary for Clinical Trial Site Readiness.” Journal of Clinical and Translational Science 7 (1): e268. https://doi.org/10.1017/cts.2023.660.
Cazier, Jean-Baptiste, Liudmila Sergeevna Mainzer, Weihao Ge, Justina Žurauskiene, and Zeynep Madak-Erdogan. 2020. “Health Disparities Research is Enabled by Data Diversity but Requires Much Tighter Integration of Collaborative Efforts.” Journal of Global Health 10, no. 2 (December). https://doi.org/10.7189%2Fjogh.10.020351.
The Center for Open Data Enterprise (CODE). 2019. Sharing and Utilizing Health Data for AI Applications: Roundtable Report. US Department of Health and Human Services. https://www.hhs.gov/sites/default/files/sharing-and-utilizing-health-data-for-ai-applications.pdf.
Centers for Disease Control and Prevention (CDC). 2023. “Health Disparities.” Updated May 26, 2023. https://www.cdc.gov/healthyyouth/disparities/index.htm.
Chaudhari, Bhushan, Himanshu Chaudhary, Aakash Agarwal, Kamna Meena, and Tanmoy Bhowmik. 2022. “FairGen: Fair Synthetic Data Generation.” Preprint, last revised December 1, 2022. https://doi.org/10.48550/arXiv.2210.13023.
Chen, Richard J., Mindy Y. Lu, Tiffany Y. Chen, Drew F. K. Williamson, and Faisal Mahmood. 2021. “Synthetic Data in Machine Learning for Medicine and Healthcare.” Nature Biomedical Engineering 5 (June): 493–497. https://doi.org/10.1038/s41551-021-00751-8.
Colón-Rodríguez, Caleb J. 2023. “Shedding Light on Healthcare Algorithmic and Artificial Intelligence Bias.” Office of Minority Health, US Department of Health and Human Services. July 12, 2023. https://minorityhealth.hhs.gov/news/shedding-light-healthcare-algorithmic-and-artificial-intelligence-bias.
Daneshjou, Roxana, Kailas Vodrahalli, Roberto A. Novoa, Melissa Jenkins, Weixin Liang, Veronica Rotemberg, Justin Ko, et al. 2022. “Disparities in Dermatology AI Performance on a Diverse, Curated Clinical Image Set.” Science Advances 8 (31). https://doi.org/10.1126/sciadv.abq6147.
Du Bois, W. E. B. (1899) 2023. The Philadelphia Negro: A Social Study. Philadelphia: University of Pennsylvania Press.
Ghosheh, Ghadeer, Jin Li, and Tingtin Zhu. 2022. “A Review of Generative Adversarial Networks for Electronic Health Records: Applications, Evaluation Measures and Data Sources.” Preprint, last revised December 14, 2022. https://doi.org/10.48550/arXiv.2203.07018.
Giuffrè, Mauro, and Dennis L. Shung. 2023. “Harnessing the Power of Synthetic Data in Healthcare: Innovation, Application, and Privacy.” npj Digital Medicine 6. https://doi.org/10.1038/s41746-023-00927-3.
Goasduff, Laurence. 2022. “Is Synthetic Data the Future of AI? Q&A with Alexander Linden.” Gartner, June 22, 2022. https://www.gartner.com/en/newsroom/press-releases/2022-06-22-is-synthetic-data-the-future-of-ai.
Gonzales, Aldren, Guruprabha Guruswamy, and Scott R. Smith. 2023. “Synthetic Data in Health Care: A Narrative Review.” PLOS Digital Health 2 (1): e0000082. https://doi.org/10.1371/journal.pdig.0000082.
Goodfellow, Ian, Jean Pouget-Abadie, Mehdi Mira, Bing Xu, David Warde-Farley, Sherjil Ozair, Aaron Courville, and Yoshua Bengio. 2014. “Generative Adversarial Nets.” In Advances in Neural Information Processing Systems 27, edited by Zoubin Ghahramani, Max Welling, Corinna Cortes, Neil D. Lawrence, and Kilian Q. Weinberger, 2672–2680. San Diego, CA: Neural Information Processing Systems.
———. 2020. “Generative Adversarial Networks.” Communications of the ACM 63 (11): 139–144. https://doi.org/10.1145/3422622.
Guevara, Marco, Shan Chen, Spencer Thomas, Tafadzwa L. Chaunzwa, Idalid Franco, Benjamin Kann, Shalini Moningi, et al. 2024. “Large Language Models to Identify Social Determinants of Health in Electronic Health Records.” Preprint, last revised March 5, 2024. https://doi.org/10.48550/arXiv.2308.06354.
Hangt, Ruth S., Linda E. Fishman, and Wendy J. Evans. 1983. Blacks and the Health Professions in the 1980s: A National Crisis and a Time for Action. Association of Minority Health Professions Schools.
Ibrahim, Hussein, Xiaoxuan Liu, Nevine Zariffa, Andrew D. Morris, and Alastair K. Denniston. 2021. “Health Data Poverty: An Assailable Barrier to Equitable Digital Health Care.” The Lancet Digital Health 3, no. 4 (April): e260–e265. https://doi.org/10.1016/S2589-7500(20)30317-4.
“Improving Data Collection across the Health Care System.” 2018. Agency for Healthcare Research and Quality. Last modified May 2018. https://www.ahrq.gov/research/findings/final-reports/iomracereport/reldata5.html.
Khan, Saad M., Xiaouxan Liu, Siddharth Naath, Edward Korot, Livia Faes, Siegfried K. Wagner, Pearse A. Keane, Neil J. Sebire, Matthew J. Burton, and Alastair K. Denniston. 2021. “A Global Review of Publicly Available Datasets for Ophthalmological Imaging: Barriers to Access, Usability and Generalizability.” The Lancet Digital Health 3, no. 1 (January): e51–e66. https://doi.org/10.1016/S2589-7500(20)30240-5.
Kokosi, Theodora, and Katie Harron. 2022. “Synthetic Data in Medical Research.” BMJ Medicine 1 (1). https://doi.org/10.1136/bmjmed-2022-000167.
Laboratory for Information and Decision Systems. 2020. “The Real Promise of Synthetic Data.” MIT News, October 16, 2020. https://news.mit.edu/2020/real-promise-synthetic-data-1016.
London, Alex John.2022. “Artificial Intelligence in Medicine: Overcoming or Recapitulating Structural Challenges to Improving Patient Care.” Cell Reports Medicine 3 (5): 1–8. https://doi.org/10.1016/j.xcrm.2022.100622.
Maayan, Gilad David. 2022. “Will Synthetic Data Introduce Ethical Challenges for ML Engineers?” Towards Data Science, July 8, 2022. https://towardsdatascience.com/will-synthetic-data-introduce-ethical-challenges-for-ml-engineers-b2608139d27f.
Marchesi, Raffale, Nicolo Micheletti, Giuseppe Jurman, and Venet Osmani. 2022. “Mitigating Health Data Poverty: Generative Approaches versus Resampling for Time-Series Clinical Data.” Preprint, last revised October 26, 2022. https://doi.org/10.48550/arXiv.2210.13958.
Martineau, Kim, and Rogerio Feris. 2023. “What Is Synthetic Data?” IBM (blog), February 8, 2023. https://research.ibm.com/blog/what-is-synthetic-data.
Metcalf, Mary P., Karen Rossie, and Kimberly Workman. 2020. “Development of an Interactive, Patient Case-Based Training Tool for Medical Professional Continuing Education.” Creative Education, 11, no. 4 (April): 500–512. https://doi.org/10.4236/ce.2020.114037.
Morgan, Thomas M. 2003 “The Education and Medical Practice of Dr. James McCune Smith (1813–1865), First Black American to Hold a Medical Degree.” Journal of the National Medical Association 95. no. 7 (July): 603–614. https://www.ncbi.nlm.nih.gov/pmc/articles/PMC2594637/.
Norori, Natalia, Qiyang Hu, Florence Marcelle Aellen, Francesca Dalia Faraci, and Athina Tzovara. 2021. “Addressing Bias in Big Data and AI for Health Care: A Call for Open Science.” Patterns 2 (10). https://doi.org/10.1016/j.patter.2021.100347.
O’Neil, Lorena. 2023. “These Women Tried to Warn Us about AI.” Rolling Stone, August 16, 2023. https://www.rollingstone.com/culture/culture-features/women-warnings-ai-danger-risk-before-chatgpt-1234804367/.
Roberts, Samuel Kelton. 2009. Infectious Fear: Politics, Disease, and the Health Effects of Segregation. Studies in Social Medicine. Chapel Hill, NC: The University of North Carolina Press.
Sehgal, Rohit. 2023. “AI Needs Data More Than Data Needs AI.” Forbes, October 5, 2023. https://www.forbes.com/sites/forbestechcouncil/2023/10/05/ai-needs-data-more-than-data-needs-ai/?sh=2e8875403ed0.
Shaghaghi, Abdolreza, Raj S. Bhopal, and Aziz Sheikh. 2011. “Approaches to Recruiting ‘Hard-To-Reach’ Populations into Research: A Review of the Literature.” Health Promotion Perspectives 1, no. 2 (December): 86–94. https://doi.org/10.5681/hpp.2011.009.
Talby, David. 2023. “The Dangers of Using Synthetic Patient Data to Build Healthcare AI Models.” Forbes, May 26, 2023. https://www.forbes.com/sites/forbestechcouncil/2023/05/26/the-dangers-of-using-synthetic-patient-data-to-build-healthcare-ai-models/.
Task Force on Black and Minority Health. 1985. Report of the Secretary’s Task Force on Black and Minority Health. Washington, DC: US Department of Health and Human Services. http://resource.nlm.nih.gov/8602912.
Walcott, Rinaldo. 2020. “Data or Politics? Why the Answer Still Remains Political.” The Globe and Mail, November 17, 2020. https://www.theglobeandmail.com/canada/article-data-or-politics-why-the-answer-still-remains-political/.
Walonoski, Jason, Sybil Klaus, Eldesia Granger, Dylan Hall, Andrew Gregorowicz, George Neyarapally, Abigail Watson, and Jeff Eastman. 2020. “Synthea™ Novel Coronavirus (COVID-19) Model and Synthetic Data Set.” Intelligence-Based Medicine 1–2 (November). https://doi.org/10.1016/j.ibmed.2020.100007.
Williams, David R., and Michelle Sternthal. 2010. “Understanding Racial-ethnic Disparities in Health: Sociological Contributions.” Journal of Health and Social Behavior 51, no. S1 (March): S15–S27. https://doi.org/10.1177/0022146510383838.