Navigating Data Politics at the Heart of AI Policy: A Workshop Summary from the Data Fluencies Project
Navigating Data Politics at the Heart of AI Policy: A Workshop Summary from the Data Fluencies Project
As AI continues to dominate public attention and private investment worldwide, regulatory scrutiny of every point in the “AI stack” becomes more pressing. The data that defines many AI products differs from that of other computing technologies, requiring a new set of policy interventions. Data raises fundamental questions on the sustainability of the “bigger is better” paradigm, the worldview of the models, the standards of development, and the capturing of human preferences in feedback. Questions about regulation and policy are essential when the paradigms driving AI development incentivize the reckless and often invasive collection of data about people and communities.[1]Emily M. Bender et al., “On the Dangers of Stochastic Parrots: Can Language Models Be Too Big?” in FAccT ’21: Proceedings of the 2021 ACM Conference on Fairness, Accountability, and … Continue reading
On July 22–23, 2024, the SSRC’s Data Fluencies Project, with the support of the Just Tech and MediaWell programs, brought together an interdisciplinary group of researchers, technologists, and practitioners to address crucial questions of data politics at the heart of AI policy, with an eye toward drawing connections between conversations in policy circles and the concrete effects of the technology in local communities. In the “Drilling Down to the Data: Navigating Data Politics at the Heart of AI Policy” workshop, led by Amba Kak and Deborah Raji, 12 participants shared their work. Their discussions coalesced around four themes:
- Bias: What should appropriate safeguards against bias and discrimination look like?
- Labor: What are the working conditions for workers training in AI systems? How can workers be protected when AI systems are used to surveil and supplant human workers across industries?
- Transparency: How can AI systems be meaningfully regulated if the datasets used to train AI are proprietary?
- Scale: How can we balance the global reach of AI against the specific regional impacts that it creates?
These conversations among such a varied group of people with different backgrounds and areas of expertise not only allowed workshop participants to interrogate the AI stack across every level, but also highlighted the need for diffusing power and the importance of creating holistic knowledge in the field of AI.
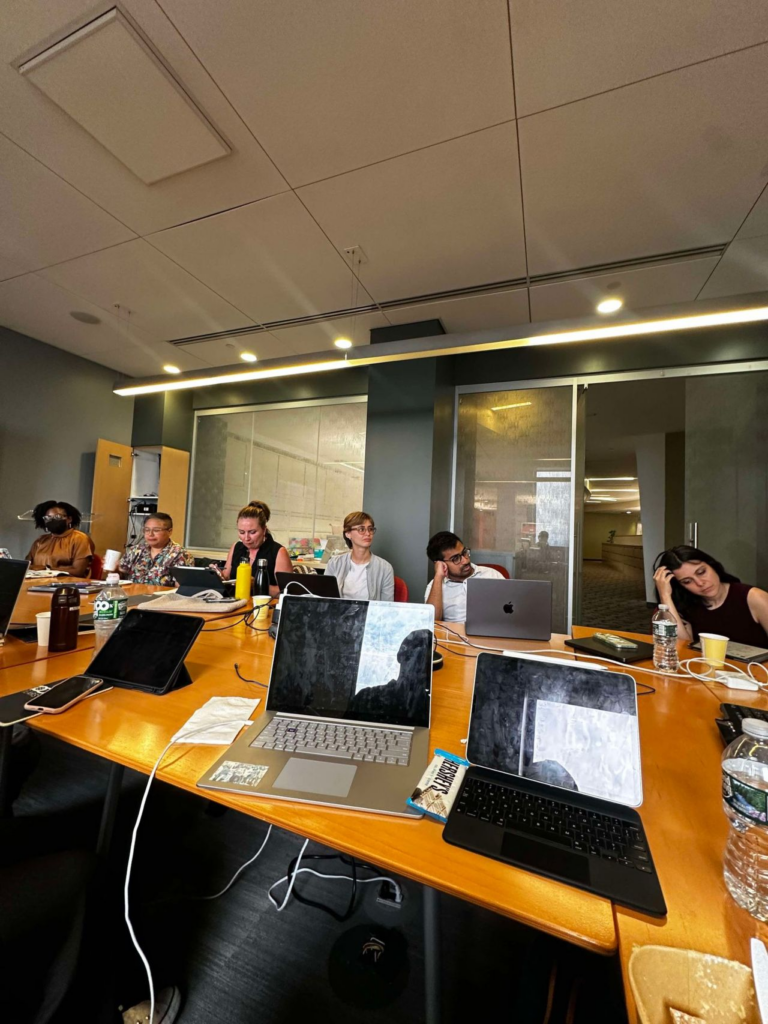
Bias
Despite the outstanding work done by researchers and practitioners over the past decade, bias problems continue to plague the development of AI technologies. Market-based solutions alone are insufficient for overcoming AI bias, and law and policymakers must take a proactive approach. This is exemplified by the work of participants Nikita Sonavane in India, Lilian Olivia Orero in Kenya, and Johann Diedrick in the United States.
Sonavane’s work emphasized the similarities between heterogeneous formations of carceral power and data-driven AI policies worldwide. She unpacked the discriminatory caste-based roots of state surveillance in India through a study of the historical precursors of digital criminal databases in Hyderabad, highlighting the transnational links of the Silicon Valley-esque formulations of data curation at the heart of AI policy in India.
Orero highlighted how data creation, collection, and deployment can perpetuate gender disparities in African countries through the misrepresentation and underrepresentation of women in AI training data. This is particularly troubling because women are often kept out of AI and tech industries by various gatekeeping mechanisms, which further exacerbates the inequitable distribution of AI’s potential benefits and harms.
Diedrick outlined a history of bias in automated speech recognition systems, highlighting how these systems discipline speech with discriminatory outcomes across race, gender, and ability, rendering speech recognition technologies difficult or impossible to access for many would-be users. Diedrick’s work addressed this bias, envisioning policy recommendations and technical specification documents for building a justice-oriented automated speech recognition system.
Labor
Human labor is foundational to the creation and maintenance of AI systems. As AI systems become ever more deeply entwined with labor, it has been shaping and disciplining the workforce in numerous ways. The work of participants Julian Posada on data workers in Venezuela, Dorothy Santos on Filipino call center workers, and Matt Canute on proprietary algorithmic systems for monitoring workers show the importance of substantive engagement with labor questions in contemporary AI policy discussions.
Posada drew on his research with data workers in Venezuela to critique data extractivism and the colonialist practices fueling the artificial intelligence industry. While some of the problems that data work generates may seem like new challenges to the rights and dignity of workers, Posada’s work underscored that these challenges must be understood in light of the persistence of outsourced labor in capitalism’s history.
Santos focused on Filipino call center workers tasked with performing “Western vocal drag” with AI-enabled assistance to critique how current AI voice recognition and accent reduction technologies are designed to devalue and eradicate foreign-accented speech in favor of hegemonic forms of English. Santos’s work pushed us to imagine how AI technologies of both voice recognition and labor discipline might be decolonized.
Canute offered an in-depth case study in which his team reproduced a corporate white paper on retail employee burnout with publicly available algorithms and data, analyzing the use of sentiment analysis technologies to monitor employee well-being in corporate environments. This method of algorithm auditing provided a model for combating the purposeful obscurity surrounding black-box proprietary algorithmic systems intended to monitor workers.
Transparency
The datasets used to train AI models are typically proprietary and not subject to public inquiry. This creates barriers to answering critical technical and governance questions about AI systems, hindering public accountability and regulatory efforts. Workshop participants Courtney Radsch, Nik Marda, and Esme Harrington interrogated this problem by examining how datasets are first compiled, outlining definitions of and best practices for open datasets and advocating for increased access for external researchers to provide accountability.
Radsch reported on the problems that the AI revolution is creating in journalism. Big tech companies are building their datasets by acquiring media companies as content sources and scraping as much high-quality human-created data as possible. These intensive data extraction practices threaten our information ecosystem, the digital economy, and the public good by making publicly available information increasingly less reliable and more subject to corporate pressures.
Marda thoroughly explored what it means for AI data to be “open,” outlining the challenges of navigating both the definitional and execution aspects of open datasets. His work provided practical recommendations for sourcing, curating, governing, and releasing these open datasets in ways that foster fair competition, enable diverse participation in AI development, and implement more fair and equitable data governance principles.
Harrington examined the status quo of external researcher access among several leading closed foundation model companies to map each company’s current access initiatives, enforcement and appeals processes, and vulnerability reporting programs. She outlined policy recommendations to improve independent research into foundation models, including creating a structured researcher access program housed in an independent intermediary body.
Scale
The research presented in this workshop centers on specific narratives about the effects of AI and datafication on several communities worldwide, connecting local and global scales of relevance. These narratives are essential to effective policymaking because they highlight what’s at stake for people on the ground. Workshop participants Jay Cunningham, Chinasa T. Okolo, and Tawana Petty showed how comparing technological and social processes from across the globe can yield surprising and valuable insights.
Cunningham’s work explored the potential of legal and technological frameworks to facilitate collective data ownership and outlines a participatory proposal to empower marginalized communities through community-centered data governance. He combined technical expertise and policy analysis to envision AI systems that prioritize societal good, reflect community values, and mitigate potential algorithmic harms.
Okolo provided an overview of data policy in African Union member states and outlines the sociopolitical infrastructure required to bolster data governance capacity across the continent. She introduced the RICE framework (reform, integration, cooperation, enforcement) as a concrete set of steps to inform the development and implementation of context-specific AI regulation that centers on data privacy rights.
Petty interrogated the impact of dominant negative narratives on shaping policy for Detroit, Michigan, which has been used as a testing ground for surveillance capitalism projects. She emphasized the problems when an environmentally challenged city becomes a testing ground for intensive data extraction and surveillance technologies.
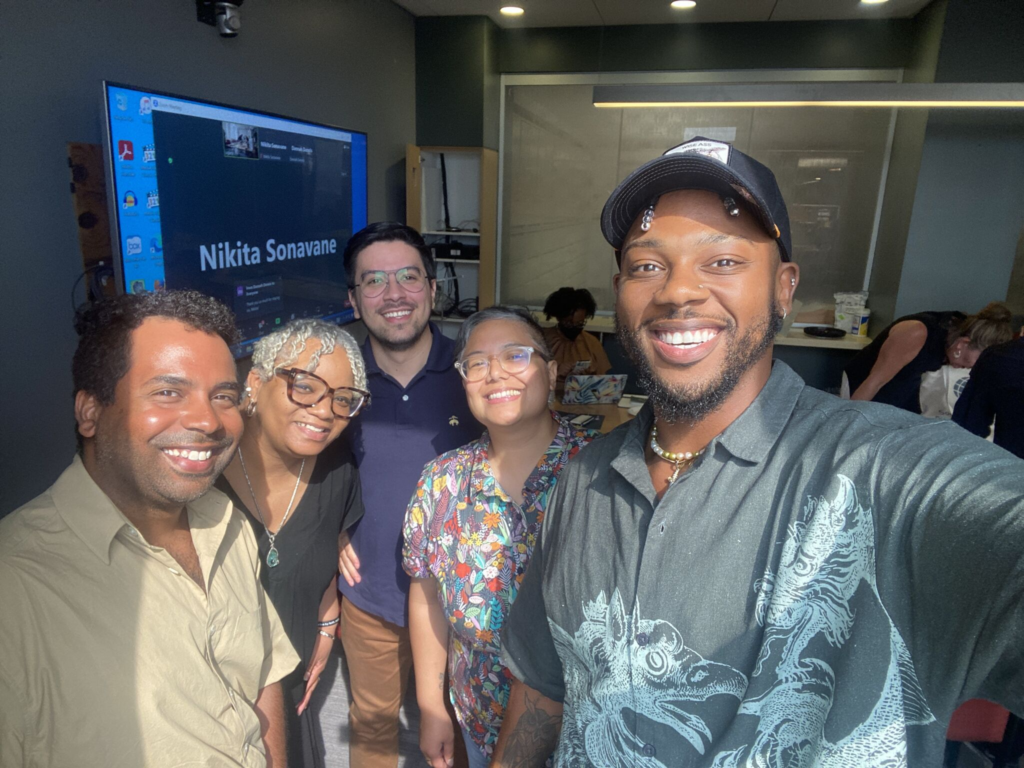
Concluding Thoughts
This workshop presented important insights into a critical overhaul of AI and data policy. First, participants critiqued corporate and state power, highlighting the need for diffusing power through the involvement of independent oversight bodies, community-led organizations, and organized labor representation. For example, Matt Canute offered a model for how independent researchers can study corporate data sets by replicating them on the basis of publicly available information, and Jay Cunningham highlighted community-based models of collective data ownership and governance.
Second, cochairs Kak and Raji emphasized the importance of creating holistic knowledge about data production and its consequences through sustained interdisciplinary conversation and collaboration. This workshop provided a valuable model for holistic knowledge creation by integrating research across the scale of the problem, from hyperlocal narratives about data creation and collection in local communities to discussions of policies that would change the existing structures of data oversight and governance on a global scale. For instance, Tawana Petty interrogated the impacts of facial recognition technologies and surveillance in a hyperlocal space such as Black communities in Detroit, and Esme Harrington advocated for a fundamental shift toward accountability for tech corporations by institutionalizing independent researcher access to corporate foundation models.
New approaches to AI policy require policymakers, researchers, practitioners, and activists to consider how bias, labor, transparency, and scale interact with data. The workshop exemplified how these dynamics manifest beyond the insularity of Silicon Valley in marginalized communities, transnational workforces, and varied institutions. Yet, the work presented demonstrates the continued need for more research to better improve the policy around AI and data.
Footnotes
↑1 | Emily M. Bender et al., “On the Dangers of Stochastic Parrots: Can Language Models Be Too Big?” in FAccT ’21: Proceedings of the 2021 ACM Conference on Fairness, Accountability, and Transparency (New York: Association for Computing Machinery, 2021), 610–623; Joy Buolamwini, Unmasking AI: My Mission to Protect What Is Human in a World of Machines (New York: Random House, 2023); Kate Crawford, Atlas of AI: Power, Politics, and the Planetary Costs of Artificial Intelligence (New Haven, CT: Yale University Press, 2022); Amba Kak and Sarah Myers West, 2023 Landscape: Confronting Tech Power (AI Now Institute, 2023); and Inioluwa Deborah Raji et al., “Closing the AI Accountability Gap: Defining an End-to-End Framework for Internal Algorithmic Auditing,” in FAT* ’20: Proceedings of the 2020 Conference on Fairness, Accountability, and Transparency (New York: Association for Computing Machinery, 2020), 33–44. |
---|