Framing Inequity in Health Technology: The Digital Divide, Data Bias, and Racialization
Framing Inequity in Health Technology: The Digital Divide, Data Bias, and Racialization
ABSTRACT
Since 2010, there has been an exponential growth in health data and health information technologies, such as electronic health records (EHRs), and AI-enabled medical tools. Despite the growth and investment in these technologies, they have had few positive effects on health outcomes, especially for marginalized populations. This review begins by addressing common rhetorical and ethical responses to inequities in health technologies, such as the digital divide and data bias frames. It then problematizes both approaches before proposing that examining racialization, or the creation and circulation of racial hierarchies, can contribute to a more comprehensive framework for facilitating health equity in health information technology.
Keywords: Digital Divide, Health Inequality, Digital Health Technologies, Health Data
Introduction
In addition to the tragic loss of life, the COVID-19 pandemic has provided a sobering reminder of the toll of health inequities. From the beginning of the pandemic, the virus seemed to discriminate as it moved through society along disproportionate yet familiar pathways of marginalization. These staggering disparities have prompted the Centers for Disease Control and Prevention (CDC) to declare racism “a serious public health threat” (CDC Online Newsroom 2021). The speed of the development of COVID-19 vaccines is evidence of the advancements in health technology, yet health disparities persist despite these innovations. The current digital health era is marked by genomics, electronic health records (EHRs), digital activity trackers and mobile health applications, and disease-predicting and diagnostic tools powered by artificial intelligence (AI), such as machine learning. Digital health technologies are imbued with the potential of Big Data—specifically that the increasing availability of digital information can be analyzed to reveal previously unknown connections and offer new insights into the factors that influence health and disease. The hope is that this new knowledge can help improve health for everyone, including groups with disproportionate disease burdens. However, as sociologist Ruha Benjamin has noted, despite the potential of digital health technologies, technology can be “one of the many conduits by which past forms of inequality are upgraded” (Benjamin 2016: 145).
Scholars and clinicians have raised the issue of inequality in digital health in two primary ways: (1) as a “digital divide” in health, and (2) as data bias in health technologies that can lead to inequality and inequity, especially for racial and ethnic minority groups. These frames are useful because they identify structural issues, such as unequal access to reliable high-speed internet. They also highlight how seemingly neutral or objective tools are vulnerable to racial bias. However, these frames also have limitations in helping us understand the landscape of digital health inequity. They don’t consider how data-centered technologies are implicated in racialization, defined here as the creation and maintenance of racial and ethnic hierarchies (Omi and Winant 2008). Identifying and understanding how racial hierarchies are created and maintained has been key to understanding inequities in other emerging technology domains, such as criminal justice (Benjamin 2019; Browne 2015).
Medical anthropologists have sought to understand how racialization produces and exacerbates health inequities in general (Gravlee and Sweet 2008; Netherland and Hansen 2017; Vega 2017), but there has been less work on digital health technologies. I argue that combining the racialization frame with the digital divide and data bias frames helps us better understand inequity in digital health. Doing so helps us identify how racialized difference circulates in digital health technologies. In this review, I show how attending to racialization in digital health points to different solutions for these inequities than those offered by the digital divide or data bias frames.
Inequity in Digital Health as Digital Divide
The phrase “digital divide” has been in scholarly and public use since at least 1999, and it corresponds to the widespread growth of internet technologies. The term generally refers to the gap between groups in their access to and use of information and communication technologies and the inequities that result from these gaps. In health, the notion of a digital divide has also been invoked to describe differences between groups in their access to and use of various digital health tools, and researchers have linked these differences to health disparities.
The “digital divide” refers to the gap between groups in their access to and use of information and communication technologies and the inequities that result from these gaps.
As early as 2005, researchers noted a digital divide in the use of new electronic health (e-health) tools like internet-enabled prescription refills and appointment scheduling. For example, researchers observed that those who were nonwhite and those who lived in low socioeconomic status neighborhoods were less likely to use e-health technologies (Hsu et al. 2005). In the United States, the 2009 HITECH Act boosted the development of e-health and digital health technologies by incentivizing healthcare organizations and providers to increase their use of EHRs (Office for Civil Rights 2009). Although this legislation led to an exponential growth in the use of EHRs, African Americans are less likely than other groups to register for newly available digital health records (Roblin et al. 2009). Racial and ethnic minorities are also less likely to use digital patient portals that provide information from digital medical records (Elkefi et al. 2021). Research has also shown that African American people are less likely than white people to post in online health discussion boards (Case et al. 2009). Scholars have also analyzed differences in how groups use mobile health, or mHealth technologies, accessed via mobile phone apps or SMS (text messages). There is some research showing that lower engagement with mHealth is connected with conditions such as hypertension (high blood pressure), and there are disparities between Black and Latina women in their use of SMS messaging and internet, which could affect their use of digital health interventions (Chilukiri et al. 2015; Langford et al. 2019). These differences in the use of digital health tools are important because using these technologies may help mitigate health disparities. For example, there is some evidence that groups with a disproportionate disease burden may benefit from using personal health records (Jackman et al. 2018) and that nonwhite patients find the ability to read their doctors’ notes in their EHR to be very important for recalling their health plan (Gerard et al. 2018).
Recently, the increasing use of telehealth during the COVID-19 pandemic has illustrated a gap in use of this technology between racial and ethnic minority groups in the United States. For example, a recent article by Rumi Chunara and colleagues (2020) shows that although Black patients are increasingly using telemedicine in New York City, they still use this technology at lower rates than do white people, and the Black patients who seek care via telemedicine are more likely to be positive for COVID-19 than are white people. Black and Hispanic patients are also more likely to get care for COVID-19 in emergency rooms or offices than via telehealth (Weber et al. 2020). Racial and ethnic minority groups in the United States have experienced disproportionately higher rates of COVID-19 infection and death, and these groups are at a disadvantage in accessing virtual care (Centers for Disease Control and Prevention 2020).
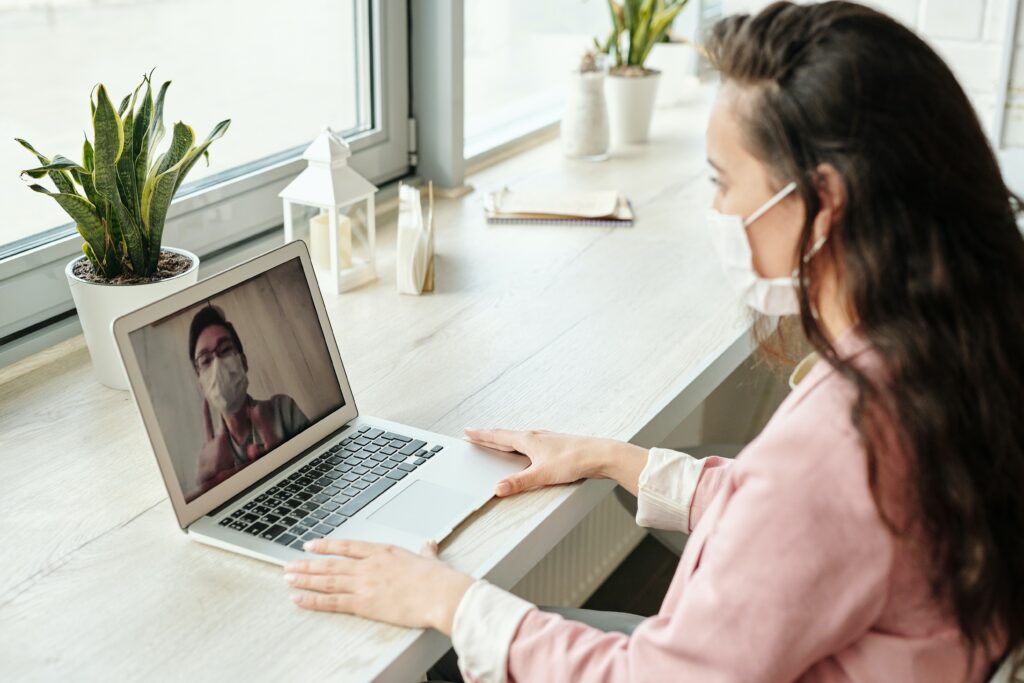
Inequality and Data Bias in New Health Technologies
In addition to the digital divide frame, inequity in health technology has been discussed using what I call the “data bias” frame. This framing focuses on how problems with data used for health technologies can lead to or exacerbate health inequities. The data bias frame examines characteristics of data, such as what is missing or excluded; the difficulties in sharing, linking, and harmonizing digital health data; and errors made because of a lack of data contexts.
Missing Data
Missing data becomes problematic and leads to health inequities, often for already marginalized groups. For example, there is significant research demonstrating the limits of genomic data, specifically that genomics studies are not representative and draw on mostly European samples. A 2016 article titled “Genetic Misdiagnoses and the Potential for Health Disparities” shows how a lack of diversity in genomic sequencing participants can lead to health inequities. The article describes a case where Black patients were mistakenly told that they carried a genetic marker for a heart condition. This marker was later shown to have been misclassified because the studies that were done to establish it as related to a heart condition did not include sufficient numbers of Black participants (Manrai et al. 2016). Due to a lack of diversity in data, these patients were given erroneous health information, were subjected to unnecessary follow-up testing, and likely experienced emotional distress. Although the diversity of genomic samples has increased, people of European descent still make up the majority in genome-wide association studies that are used to explore the relationships between genetics and disease (Devaney 2019; Roberts, Khoury, and Mensah 2019). Scholars have argued that increasing the diversity of genomics data sets is key for ensuring that the use of genomics in medicine advances health equity and does not exacerbate health disparities (Jooma et al. 2019).
Racial and ethnic minority groups also seem to be missing from EHR data, which can lead to bias if these data are used in digital health applications. There are some US state and federal policies that incentivize the collection of demographic information (such as race and ethnicity) in EHRs, and some have argued that collecting this information is important because it can be used to address racial and other disparities in health (Chin 2015; Zhang et al. 2017). However, some patients, including those from minoritized groups, have been reluctant to provide demographic information when asked in healthcare settings (Chin 2015). Clinical staff have expressed doubts that the mere collection of racial identity information and other demographic data will lead to substantial mitigations of health disparities (Cruz and Smith 2021; Pérez-Stable, Jean-Francois, and Aklin 2019). But this data “missingness” or “data absenteeism” in EHRs is seen as problematic for those developing AI health tools because it makes them vulnerable to “pernicious bias” that can disproportionally harm marginalized groups (Ghassemi et al. 2020; Lee and Viswanath 2020; McCradden et al. 2020).
The logic of the data bias frame is that AI tools become biased because of lack of representative data, and this adds to patterns of health inequity.
Fragmented EHR data, which results when individuals seek care from multiple healthcare providers over time, also can be a source of bias. Researchers often prefer to work with digital medical records that are more complete and feature long-term care relationships with providers and health systems (Ferryman and Pitcan 2018; West et al. 2014). Scholars have also noted that in the US, Hispanic and Asian/Pacific Islander populations are more likely than white people to have missing linkages between Social Security numbers (SSN) and death certificates. This discrepancy is explained by the fact that SSN information for these populations is more often missing from National Health Interview Survey (NHIS) data, “either due to [individuals] not having it, not knowing it, refusing to provide it, or not being asked” (Miller, McCarty, and Parker 2017, 79). The logic of the data bias frame is that AI tools become biased because of lack of representative data, and this adds to patterns of health inequity.
In some cases, digital health data may not be representative, as in the previous examples. But in other instances, data from racial and ethnic groups may be present but obscured, which can lead to these groups’ apparent absence from the data. For example, Jennifer Young and Mildred Cho (2021) argue that the category of “Asian American” is too broad and obscures not only ethnic variation but important health disparities. Karen Wang and colleagues (2020) have shown that differing racial and ethnic categories in health data pose challenges when these data sets are brought together for analysis. Harmonizing health data thus results in mixed-race people being recategorized or left out, and subpopulation and subgroup nuance is lost when data are reordered and pooled into larger racial and ethnic categories. They describe this as “data privilege,” where some people are represented in the data and some are not because of the way categories are constructed (Wang et al. 2020).
Lack of Data Context and Empathy
Digital health tools can exhibit pernicious bias because the people who build these tools may lack knowledge about the data’s meanings or origins. This has been referred to as a lack of “data empathy” (Ferryman and Pitcan 2018) or “data chauvinism,” which refers to “faith in the size of the data without considerations for quality and contexts” (Lee and Viswanath 2020). Missteps can occur when data are used in algorithmic and AI health applications, as was evident in an algorithmic model that predicted healthier white patients were more in need of health resources than were sicker Black patients (Obermeyer et al. 2019). In this example, the problem was not missing or obscured data. The problem was that the healthcare institution overlooked important information about the meaning and context of the data. The algorithm used healthcare expenditure as a proxy for identifying a person’s level of health: more money meant that the patient was sicker, while less money spent by the institution on the patient meant that the patient was healthier. However, using expenditure as a proxy missed the context of medical discrimination: typically, healthcare institutions spend less money on Black patients even when they are sicker.
There has been growing interest in social media data as a source of health data for underrepresented groups and as a source of information on health issues where there is underreporting, such as miscarriage (Cesare et al. 2020; Zhao et al. 2020). When subjected to AI analysis, social media data can also be vulnerable to bias from analysts’ lack of knowledge and context. For example, Brandon Lwowski and Anthony Rios (2021) show that machine-learning models that use tweets in standard English may miss relevant health information shared in tweets using African American Vernacular English.
Although inequity in genomics and other forms of health data can spring from a lack of representativeness (as the data bias frame emphasizes), pernicious bias can emerge even when racial and ethnic minority groups are sufficiently represented. Patterns of health inequity and marginalization can be created and reproduced because of a lack of understanding of data context and limited data empathy.
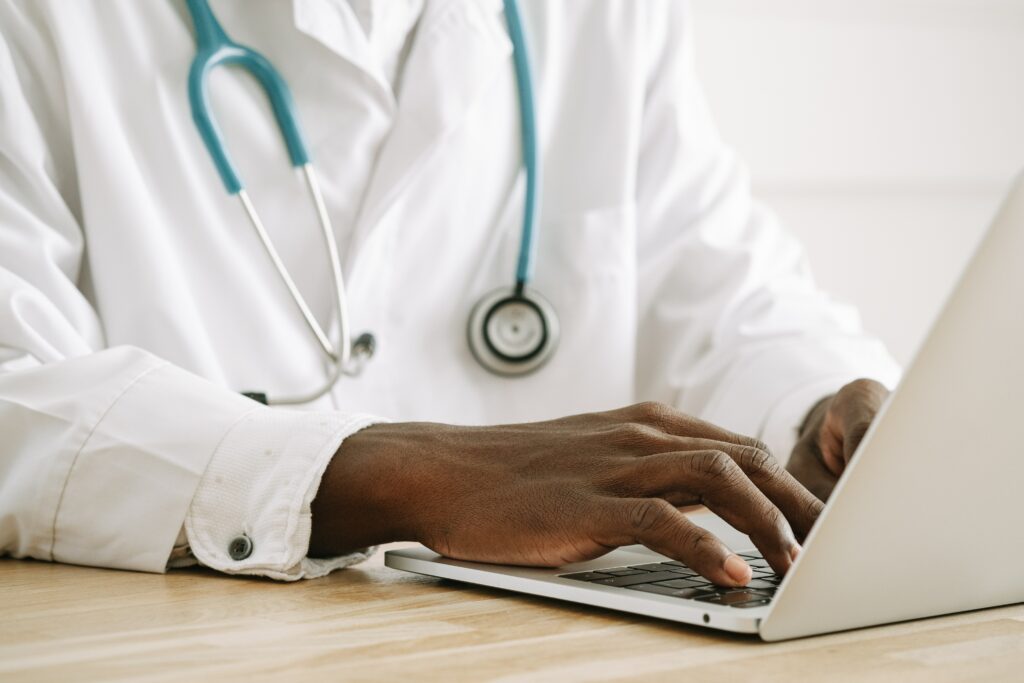
Problems with These Frames
The digital divide and data bias frames outline important dimensions of inequality and inequity in digital health. However, because these are conceptual tools that help structure our understanding, when we rely on them we often can only see specific problems and solutions, and not others.
For example, the digital divide frame suggests equalizing access and use of digital health technologies among racialized minority groups. If lack of engagement with online health tools is the problem, then removing access barriers (such as providing better internet access) would be a solution. The power of the digital divide frame in connecting health disparities to lack of access to internet technology is evident in US Department of Health and Human Services Secretary Xavier Becerra’s comments about President Joe Biden’s proposed infrastructure development plan. Becerra links health inequity to a lack of infrastructure and remarks that expanding broadband internet access is key to addressing health disparities (Hendel and Ravindranath 2021).
Despite the positive effects that may come from equalized internet access, this focus on racialized minority groups’ lack of engagement with digital health technologies is limiting.
Despite the positive effects that may come from equalized internet access, this focus on racialized minority groups’ lack of engagement with digital health technologies is limiting. Daniel Lorence, Heeyoung Park and Susannah Fox (2008) have argued “that given the persistence of a digital divide for health consumers, there is a need to examine distinctions within these digitally under-served groups, using targeted strategies tailored to the needs of subpopulations, rather than attempting to categorize the digital gap as a single entity” (30). In other words, we need to shift from thinking about a single digital divide in health to thinking about multiple divides and the distinct reasons these gaps emerge in different subpopulations.
Along similar lines, LaPrincess Brewer and colleagues argue that this digital divide should be viewed as “a paucity of culturally informed or culturally useful health informatics or digital health interventions” rather than just driven by lack of technology access and use (Brewer et al. 2020: 2). Thus, the issue may not be a lack of access or engagement; instead, e-health tools themselves might be insufficient.
Other researchers have emphasized that the digital divide frame overemphasizes deficits, such as a lack of e-health literacy. Instead of characterizing people as information-poor, we could discuss individuals in terms of their “information capability.” This reframing recognizes that “people have a greater or lesser need for information . . . [and a] greater or lesser awareness of its usefulness or applicability [and] the ability to exploit information once it has been acquired” (McPherson and Wilkinson 2001, 64). These authors point out that there may be variation in information capability in cultures and subcultures, which reinforces the idea that there are many digital divides rather than a single one. Courtney Lyles and colleagues find that some African Americans and Latinos are open to the idea of digital patient portals but also have a “fear of the portal eroding existing personal relationships with health care providers” (Lyles et al. 2016: 1). Again, this suggests that patients discern when and how they want to use digital health technologies, not that they lack e-health literacy. Furthermore, some recent research suggests that the digital divide may not be that large of a gap, as there is little difference between groups in their use of digital health tools overall (Nouri et al. 2020). In addition, the high levels of ownership of smartphones in racial and ethnic minority groups can be seen as an opportunity or advantage for engaging with digital health technologies. For example, text messaging health information and reminders for follow-up care has been useful for populations experiencing health disparities, such as African Americans in the United States who have higher rates of cervical cancer (Romocki et al. 2019).
Because the digital divide frame focuses on particular barriers, it also leads to particular solutions. Although education and more access to broadband internet are important interventions, they do not address broader social conditions. For instance, residential segregation produces differential access to high-speed internet or and the limitations of digital health tools may explain different levels of engagement.
The data bias frame also suggests particular solutions. In genomics, a reasonable solution to the bias toward European samples would be to make the data more diverse, representative, and inclusive. However, as legal scholar Shawneequa Callier (2019) cautions, making genomic data sets more diverse can be a complex endeavor. It matters which categories are used. For example, metrics of achieving sufficient genetic diversity in samples could change if US census racial and ethnic categories were used instead of racial categories from other countries or measures of ancestral admixture (Callier 2019).
Making data more representative and diverse is also offered as a solution for digital and algorithmic health tools. For example, Rahuldeb Sarkar and colleagues (2021) find that models overpredict mortality for Black and Latino patients. They warn that these models should be used cautiously for triaging people who seek care at hospitals, especially during the COVID-19 pandemic. They propose updating these models with more real-time data and diversifying the groups on which models are tested, so that these kinds of overestimates can be accounted for (Sarkar et al. 2021). In other cases, such as the algorithmic model favoring healthier white patients over sicker Black patients, more diverse data would not help. Knowing more about the data, such as its embedded biases, would prevent some models from repeating patterns of discrimination. However, these solutions are limited to the realm of data itself, like “fixing” the data by making it more diverse.
Knowing more about the data, such as its embedded biases, would prevent some models from repeating patterns of discrimination.
More inclusive data sets may not fix the discrimination in digital health tools. As Nikki Stevens and Os Keyes argue, we should not just focus on “biased datasets” because “the datasets themselves are not so much biased as they are reflective of their sites of use” (2021, 16). They go on to say that we must instead focus on the “logics and systems of inequality that lead to the datasets’ purposes, and so naturalize the datasets’ demographic skews” (16).
Genomics and other digital health data sets are not biased by accident. They mirror societal processes of marginalization that go beyond discrete or incomplete data sets. Flawed data sets can be fixed, but other biased data sets may continue to be identified and created. Data have histories and reflect researchers’ explicit choices about what they consider important questions to be asked, what they deem important information to be collected, and how they will measure that information. Several questions can follow from this kind of inquiry: Why do data sets and the models built on them continue to privilege dominant groups? When models are found to be replicating patterns of marginalization and discrimination, one common refrain is “garbage in, garbage out,” or that bad data is going to result in a bad model. But that “garbage data” may not need to be discarded, as they are artifacts that reflect political arrangements and systems of inequality, such as racial hierarchies (Winner 1980).
Another Frame: Racialization
I propose a third frame for understanding inequity in digital health: racialization, or the processes by which racial hierarchies are created, circulated, and maintained. These shifting hierarchies structure who is assumed to count as “human” and thus affect our life experiences in multiple domains. Understanding inequity in health through the frame of racialization allows us to see different problems and solutions than those made visible by the frames of digital divide or data bias.
Medical anthropologists have used racialization as a way to explain inequities in health. Khiara Bridges (2011) argues that Black women are racialized when they seek prenatal care. Clinicians racialize them by viewing them as uneducated and “wily” patients who are out to game the healthcare system (Bridges 2011). Racial hierarchies also shape clinical views of disease. Julie Netherland and Helena Hansen (2017) demonstrate that opioid use is criminalized when Black people are addicted but treated as a clinical condition worthy of sympathy and therapeutic intervention when the users are white. Additionally, Daisy Deomampo (2019) has noted that racial hierarchies shape the demand and market for donor eggs in assisted reproduction.
The racialization frame provides another way of understanding inequity in digital health and can address the gaps left by the digital divide and data bias frames.
The racialization frame provides another way of understanding inequity in digital health and can address the gaps left by the digital divide and data bias frames. For example, Darshali Vyas, Leo Eisenstein, and David Jones (2020) identify how clinical risk calculators are modified, or based on an individual’s race and argue that these so-called racial corrections are sometimes invisible to clinicians and patients. Some corrections are based on racial stereotypes about innate or biological differences between groups, such as the correction made to a kidney function calculator to adjust for supposedly more muscular Black bodies or the correction made in lung function measurements to adjust for the purportedly smaller lungs of Black and Asian people (Balch 2020). These seemingly objective corrections have limited or questionable clinical research supporting them and instead draw on stereotypes and white supremacist hierarchies of racial groups. These clinical racial corrections are dangerous because they often invisibly reinforce these racial categorizations and because they often inaccurately lower health risks for racialized minority groups. These corrections can lead to undertreatment and less diagnostic and preventative care.
We could think of racial corrections used in clinical algorithms as a data bias problem, since the “corrected” risk score is biased against racial and ethnic minority groups. However, these racial corrections may not be fixed by including more diverse data. The items included in the calculators, like blood pressure or race, may not be biased or incorrect. Another solution might be to leave race out of clinical risk algorithms, but that introduces other problems. Harald Schmidt, Dorothy Roberts, and Nwamaka Eneanya’s (2021) examination of ventilator priority shows that the effect of race in risk calculators may be present even when race is excluded as a variable in these calculators. New Jersey’s ventilator distribution guidelines draw on a risk calculator called the Sequential Organ Failure Assessment (SOFA) and combines it with another measure of survival in the near term. Together, these scores prioritize those who would most benefit from a ventilator because their chances of recovery in the short term are higher and they have an overall longer life expectancy. On the surface, this seems to be a reasonable way to determine priority for a scarce clinical resource, but the authors argue that this system unjustly deprioritizes Black people. This is because SOFA includes creatinine, as higher creatinine levels can indicate kidney disease. However, creatinine is not just a physiological measure and SOFA ignores the “social disadvantages that may cause higher creatinine” (Schmidt, Roberts, and Eneanya 2021, 2). Higher levels of creatinine result from chronic conditions such as kidney disease and high blood pressure. The prevalence of these conditions in racial and ethnic minority groups can be “best understood as the consequences of health inequities and structural racism” (Schmidt, Roberts, and Eneanya 2021, 2).
Here we see racialization at work. Racial hierarchies structure social opportunities, and social opportunities affect health. For racialized minorities, these health effects include disproportionate rates of chronic diseases that are not due to genetic differences or lifestyle choices alone (Roberts 2012). Thus, the health data used in these calculators reflect histories of racialization and reproduce patterns of racial marginalization so that Black people are more likely to receive a lower ventilator priority score. In this example, the data could be “fixed” by removing creatinine as a measure in this risk calculator. However, as noted already, there are multiple racial corrections used clinically, which are then included in other technologies, like risk assessment mobile health apps. When this kind of racial bias is so prevalent in medicine, trying to root out bias is a kind of Whac-A-Mole. As soon as one biased tool is identified and corrected, another one pops up.
An alternative is to use the frame of racialization to understand how racial hierarchies shape the healthcare domain and how digital data reproduce racialization. Racialization points to different kinds of solutions. For example, using the racialization frame would mean asking how racial hierarchies affect digital health tools, what histories the data reflect, how and why some groups might be marginalized or harmed by digital health technologies, and whose expertise and values are included in the development of these health tools. Although not specifically focused on digital health, Stacie Daugherty and colleagues’ (2019) research provides an illustrative example of the value of using racialization to think through marginalization in healthcare spaces. They discuss how patients can be discriminated against via stereotype threat, and this can negatively affect the care they receive as well as their health outcomes (Daugherty et al. 2019). Their research shows how values-affirming language may help with clinical outcomes, such as medication adherence. Treatment compliance is not attributed to a deficit in racialized groups but instead to processes of racialization—and these processes structure clinical experiences and disparate outcomes.
The racialization frame prompts us to consider how race is represented in digital health tools, and how and why these representations change (Benthall and Haynes 2019). This would add another dimension to discussions of algorithmic fairness and disparate effects in digital health, as groups that are nominally the same might be constructed in different ways in clinical data. In addition, when racialization processes are recognized as an important contributor to health inequities, digital health apps—such as Irth and Patient Orator, which were developed by Black women as a way to have their voices heard—can be understood as much-needed interventions to counter clinical stereotyping and discrimination.
Public health critical race (PHCR) praxis puts racialization at the forefront. As Chandra Ford and Collins Airhihenbuwa argue, this conceptual tool helps “to move beyond merely documenting health inequities toward understanding and challenging the power hierarchies that undergird them” (2010, 1390). PHCR draws on critical race theory to examine racialization and health inequities. In addition, “healthcrits,” or those using PHCR as a framework, focus on innovative ways of eliminating health inequities. Although PHCR is designed to be used in overall health research, its key tenets and principles can offer alternate inquiries and interventions in digital health. For example, a fundamental question in PHCR is to ask how racialization unfolds in the domain of interest. In digital health, this would mean asking how racialization informs health data and comprehensively examining how and why racial categories are represented the way they are. It would mean being attentive to how racial hierarchies are reflected in the data, such as in creatinine measurements.
Interventions focusing on racialization, like PHCR, involve turning a critical eye to health researchers and experts and would address the digital divide differently. Solutions would not only focus on expanding internet access and developing e-health literacy but also look more like building digital coordinated care networks, an effort facilitated by Lisa Peyton-Caire, executive director of the Foundation for Black Women’s Wellness. Peyton-Caire created an advisory group of 300 Black women to advise Epic (a major EHR provider) and a number of health systems on how to prioritize the crisis of Black women’s maternal health while serving the overall needs of the patient community by creating links between clinical institutions and community organizations. This project draws on the capacity of racialized groups, rather than perceived deficits. It also highlights how existing health data are already racialized and can disrupt the pattern of building digital health technologies that need “fixing” later on. This kind of upstream involvement in the conceptualization and design of digital health tools can help ensure that they will be attuned to racialization and its negative effects from the beginning.
Instead of focusing on marginalized groups’ deficits or technological fixes to digital artifacts, I suggest we employ the racialization frame to ask how gaps emerge and why technologies reify social distinctions and disadvantage.
This review has examined two frames that are often used to understand inequality and inequity in digital health: the digital divide and data bias. These approaches index important differences in digital health technology access and use, and they draw attention to the flaws in data that are used to develop health technologies. I have also offered the racialization frame as a complement. The racialization frame points to the myriad ways social hierarchies are reflected, shifted, and reproduced in digital health technologies. Instead of focusing on marginalized groups’ deficits or technological fixes to digital artifacts, I suggest we employ the racialization frame to ask how gaps emerge and why technologies reify social distinctions and disadvantage. Racialization orients us toward digital health development that disrupts ingrained patterns of devaluation.
Recommended Readings
Benjamin, Ruha. 2019. Race after Technology: Abolitionist Tools for the New Jim Code. Cambridge: Polity.
Brewer, LaPrincess C., Karen L. Fortuna, Clarence Jones, Robert Walker, Sharonne
N. Hayes, Christi A. Patten, and Lisa A. Cooper. 2020. “Back to the Future: Achieving
Health Equity through Health Informatics and Digital Health.” JMIR mHealth and
uHealth 8, no. 1: e14512. https://doi.org/10.2196/14512.
Chunara, Rumi, Yuan Zhao, Ji Chen, Katharine Lawrence, Paul A. Testa, Oded Nov,
and Devin M. Mann. 2020. “Telemedicine and Healthcare Disparities: A Cohort Study in a Large Healthcare System in New York City during COVID-19.” Journal of the American Medical Informatics Association 28, no. 1: 33-41. https://doi.org/10.1093/jamia/ocaa217.
Cruz, Taylor M., and Sheridan A. Smith. 2021. “Health Equity beyond Data: Health
Care Worker Perceptions of Race, Ethnicity, and Language Data Collection in Electronic Health Records.” Medical Care 59, no. 5: 379–85.
Schmidt, Harald, Dorothy E. Roberts, and Nwamaka D. Eneanya. 2021. “Rationing,
Racism and Justice: Advancing the Debate around ‘Colourblind’ COVID-19
Ventilator Allocation.” Journal of Medical Ethics, January. https://doi.org/10.1136/medethics-2020-106856
References
Balch, Bridget. 2020. “Confronting Race in Diagnosis: Medical Students Call for Reexamining How Kidney Function Is Estimated.” Association of American Medical Colleges, September 24. https://www.aamc.org/news-insights/confronting-race-diagnosis-medical-students-call-reexamining-how-kidney-function-estimated.
Braun, Lundy, Anna Wentz, Reuben Baker, Ellen Richardson, and Jennifer Tsai. 2020. “Racialized algorithms for kidney function: Erasing social experience.” Social Science & Medicine 268, no 113548: 1-9.
Benjamin, Ruha. 2016. “Catching Our Breath: Critical Race STS and the Carceral Imagination.” Engaging Science, Technology, and Society 2: 145-156.
Benjamin, Ruha. 2019. Race after Technology: Abolitionist Tools for the New Jim Code. Cambridge: Polity.
Benthall, Sebastian, and Bruce D. Haynes. 2019. “Racial Categories in Machine Learning.” In Proceedings of the Conference on Fairness, Accountability, and Transparency, 289–98. FAT* ’19, New York. Association for Computing Machinery. https://doi.org/10.1145/3287560.3287575.
Brewer, LaPrincess C., Karen L. Fortuna, Clarence Jones, Robert Walker, Sharonne N. Hayes, Christi A. Patten, and Lisa A. Cooper. 2020. “Back to the Future: Achieving Health Equity through Health Informatics and Digital Health.” JMIR mHealth and uHealth 8, no. 1: e14512. https://doi.org/10.2196/14512.
Bridges, Khiara M. 2011. Reproducing Race: An Ethnography of Pregnancy as a Site of Racialization. Berkeley: University of California Press.
Browne, Simone. 2015. Dark Matters: On the Surveillance of Blackness. Durham, NC: Duke University Press.
Callier, Shawneequa L. 2019. “The Use of Racial Categories in Precision Medicine Research.” Ethnicity & Disease 29, no. S3: 651–58. https://doi.org/10.18865/ed.29.S3.651.
Case, Siobhan, Valarie Blue Bird Jernigan, Audra Gardner, Philip Ritter, Catherine Heaney, and Kate Lorig. 2009. “Content and Frequency of Writing on Diabetes Bulletin Boards: Does Race Make a Difference?” Journal of Medical Internet Research 11, no. 2: e1153. https://doi.org/10.2196/jmir.1153.
Centers for Disease Control and Prevention (CDC). 2020. “Cases, Data, and Surveillance.” Centers for Disease Control and Prevention, February 11. https://www.cdc.gov/coronavirus/2019-ncov/covid-data/investigations-discovery/hospitalization-death-by-race-ethnicity.html.
CDC Online Newsroom. 2021. “Media Statement from CDC Director Rochelle P. Walensky, MD, MPH, on Racism and Health.” Centers for Disease Control and Prevention, April 8. https://www.cdc.gov/media/releases/2021/s0408-racism-health.html.
Cesare, Nina, Olubusola Oladeji, Kadija Ferryman, Derry Wijaya, Karen D. Hendricks‐Muñoz, Alyssa Ward, and Elaine O. Nsoesie. 2020. “Discussions of Miscarriage and Preterm Births on Twitter.” Paediatric and Perinatal Epidemiology 34, no. 5: 544–52. https://doi.org/10.1111/ppe.12622.
Chilukuri, Nymisha, Meredith West, Janice Lynn Henderson, Shari Lawson, Robert Ehsanipoor, Kathleen Costigan, Sarah Polk, and Wendy Bennett. 2015. “Information and Communication Technology Use among Low-Income Pregnant and Postpartum Women by Race and Ethnicity: A Cross-Sectional Study.” Journal of Medical Internet Research 17, no. 7: e3916. https://doi.org/10.2196/jmir.3916.
Chin, Marshall. 2015. “Using Patient Race, Ethnicity, and Language Data to Achieve Health Equity.” Journal of General Internal Medicine 30, no. 6: 703–5.
Chunara, Rumi, Yuan Zhao, Ji Chen, Katharine Lawrence, Paul A. Testa, Oded Nov, and Devin M. Mann. 2020. “Telemedicine and Healthcare Disparities: A Cohort Study in a Large Healthcare System in New York City during COVID-19.” Journal of the American Medical Informatics Association 28, no. 1: 33–41. https://doi.org/10.1093/jamia/ocaa217.
Cruz, Taylor M., and Sheridan A. Smith. 2021. “Health Equity beyond Data: Health Care Worker Perceptions of Race, Ethnicity, and Language Data Collection in Electronic Health Records.” Medical Care 59, no. 5: 379–85.
Daugherty, Stacie L., Suma Vupputuri, Rebecca Hanratty, John F. Steiner, Julie A. Maertens, Irene V. Blair, L. Miriam Dickinson, Laura Helmkamp, and Edward P. Havranek. 2019. “Using Values Affirmation to Reduce the Effects of Stereotype Threat on Hypertension Disparities: Protocol for the Multicenter Randomized Hypertension and Values (HYVALUE) Trial.” JMIR Research Protocols 8, no. 3: e12498. https://doi.org/10.2196/12498.
Deomampo, Daisy. 2019. “Racialized Commodities: Race and Value in Human Egg Donation.” Medical Anthropology 38, no. 7: 620–33. https://doi.org/10.1080/01459740.2019.1570188.
Devaney, Stephanie. 2019. “All of Us.” Nature 576 (December): S14–17. https://doi.org/10.1038/d41586-019-03717-8.
Elkefi, Safa, Zhongyuan Yu, and Onur Asan. 2021. “Online Medical Record Nonuse among Patients: Data Analysis Study of the 2019 Health Information National Trends Survey.” Journal of Medical Internet Research 23, no. 2: e24767. https://doi.org/10.2196/24767.
Ferryman, Kadija, and Mikaela Pitcan. 2018. Fairness in Precision Medicine. New York: Data and Society Research Institute.
Ford, Chandra L., and Collins O. Airhihenbuwa. 2010. “The Public Health Critical Race Methodology: Praxis for Antiracism Research.” Social Science & Medicine 71, no. 8: 1390–98. https://doi.org/10.1016/j.socscimed.2010.07.030.
Gerard, Macda, Hannah Chimowitz, Alan Fossa, Fabienne Bourgeois, Leonor Fernandez, and Sigall K. Bell. 2018. “The Importance of Visit Notes on Patient Portals for Engaging Less Educated or Nonwhite Patients: Survey Study.” Journal of Medical Internet Research 20, no. 5: e9196. https://doi.org/10.2196/jmir.9196.
Ghassemi, Marzyeh, Tristan Naumann, Peter Schulam, Andrew L. Beam, Irene Y. Chen, and Rajesh Ranganath. 2020. “A Review of Challenges and Opportunities in Machine Learning for Health.” AMIA Summits on Translational Science Proceedings 2020 (May): 191–200.
Gravlee, Clarence C., and Elizabeth Sweet. 2008. “Race, Ethnicity, and Racism in Medical Anthropology, 1977–2002.” Medical Anthropology Quarterly 22, no. 1: 27–51. https://doi.org/10.1111/j.1548-1387.2008.00002.x.
Hendel, John, and Mohana Ravindranath. 2021. “Biden’s Health Equity Efforts Start with Broadband Push.” Politico, March 31. https://politi.co/3cEMQVI.
Hsu, John, Jie Huang, James Kinsman, Bruce Fireman, Robert Miller, Joseph Selby, and Eduardo Ortiz. 2005. “Use of E-Health Services between 1999 and 2002: A Growing Digital Divide.” Journal of the American Medical Informatics Association 12, no. 2: 164–71. https://doi.org/10.1197/jamia.M1672.
Jackman, Kevon-Mark, Stefan David Baral, Lisa Hightow-Weidman, and Tonia Poteat. 2018. “Uncovering a Role for Electronic Personal Health Records in Reducing Disparities in Sexually Transmitted Infection Rates among Students at a Predominantly African American University: Mixed-Methods Study.” JMIR Medical Informatics 6, no. 3: e9174. https://doi.org/10.2196/medinform.9174.
Jooma, Sonya, Michael J. Hahn, Lucia A. Hindorff, and Vence L. Bonham. 2019. “Defining and Achieving Health Equity in Genomic Medicine.” Ethnicity & Disease 29, no. S1: 173–78. https://doi.org/10.18865/ed.29.S1.173.
Langford, Aisha T., Craig A. Solid, Ebony Scott, Meeki Lad, Eli Maayan, Stephen K. Williams, and Azizi A. Seixas. 2019. “Mobile Phone Ownership, Health Apps, and Tablet Use in US Adults with a Self-Reported History of Hypertension: Cross-Sectional Study.” JMIR mHealth and uHealth 7, no. 1: e12228. https://doi.org/10.2196/12228.
Lee, Edmund W. J., and Kasisomayajula Viswanath. 2020. “Big Data in Context: Addressing the Twin Perils of Data Absenteeism and Chauvinism in the Context of Health Disparities Research.” Journal of Medical Internet Research 22, no. 1: e16377. https://doi.org/10.2196/16377.
Lorence, Daniel P., Heeyoung Park, and Susannah Fox. 2006. “Racial Disparities in Health Information Access: Resilience of the Digital Divide.” Journal of Medical Systems 30, no. 4: 241–49. https://doi.org/10.1007/s10916-005-9003-y.
Lwowski, Brandon, and Anthony Rios. 2021. “The Risk of Racial Bias While Tracking Influenza-Related Content on Social Media Using Machine Learning.” Journal of the American Medical Informatics Association 28, no. 4: 839–49. https://doi.org/10.1093/jamia/ocaa326.
Lyles, Courtney Rees, Jill Y. Allen, Dolly Poole, Lina Tieu, Michael H. Kanter, and Terhilda Garrido. 2016. “‘I Want to Keep the Personal Relationship with My Doctor’: Understanding Barriers to Portal Use among African Americans and Latinos.” Journal of Medical Internet Research 18, no. 10: e5910. https://doi.org/10.2196/jmir.5910.
Manrai, Arjun K., Birgit H. Funke, Heidi L. Rehm, Morten S. Olesen, Bradley A. Maron, Peter Szolovits, David M. Margulies, Joseph Loscalzo, and Isaac S. Kohane. 2016. “Genetic Misdiagnoses and the Potential for Health Disparities.” New England Journal of Medicine 375, no. 7: 655–65. https://doi.org/10.1056/NEJMsa1507092.
McCradden, Melissa D., Shalmali Joshi, James A. Anderson, Mjaye Mazwi, Anna Goldenberg, and Randi Zlotnik Shaul. 2020. “Patient Safety and Quality Improvement: Ethical Principles for a Regulatory Approach to Bias in Healthcare Machine Learning.” Journal of the American Medical Informatics Association 27, no. 12: 2024–27. https://doi.org/10.1093/jamia/ocaa085.
McPherson, S., and M. Wilkinson. 2001. “Health Informatics and the Cultural Divide: A UK Perspective.” Health Informatics Journal 7, no. 2: 62–65. https://doi.org/10.1177/146045820100700202.
Miller, Eric A., Frances A. McCarty, and Jennifer D. Parker. 2017. “Racial and Ethnic Differences in a Linkage with the National Death Index.” Ethnicity & Disease 27, no. 2: 77–84. https://doi.org/10.18865/ed.27.2.77.
Netherland, Julie, and Helena Hansen“White Opioids: Pharmaceutical Race and the War on Drugs That Wasn’t.” BioSocieties 12: 217–38. https://doi.org/10.1057/biosoc.2015.46.
Nouri, Sarah S., Julia Adler-Milstein, Crishyashi Thao, Prasad Acharya, Jill Barr-Walker, Urmimala Sarkar, and Courtney Lyles. 2020. “Patient Characteristics Associated with Objective Measures of Digital Health Tool Use in the United States: A Literature Review.” Journal of the American Medical Informatics Association 27, no. 5: 834–41. https://doi.org/10.1093/jamia/ocaa024.
Obermeyer, Ziad, Brian Powers, Christine Vogeli, and Sendhil Mullainathan. 2019. “Dissecting Racial Bias in an Algorithm Used to Manage the Health of Populations.” Science 366, no. 6464: 447–53. https://doi.org/10.1126/science.aax2342.
Office for Civil Rights. 2009. HITECH Act Enforcement Interim Final Rule. https://www.hhs.gov/hipaa/for-professionals/special-topics/HITECH-act-enforcement-interim-final-rule/index.html.
Omi, Michael, and Howard Winant. 2008. “Once More, with Feeling: Reflections on Racial Formation.” PMLA 123, no. 5: 1565–72.
Pérez-Stable, Eliseo J., Beda Jean-Francois, and Courtney Ferrell Aklin. 2019. “Leveraging Advances in Technology to Promote Health Equity.” Medical Care 57, no. 6: S101–3.
Roberts, Dorothy. 2012. “Debating the Cause of Health Disparities: Implications for Bioethics and Racial Equality.” Cambridge Quarterly of Healthcare Ethics 21: 332–41.
Roberts, Megan C., Muin J. Khoury, and George A. Mensah. 2019. “Perspective: The Clinical Use of Polygenic Risk Scores: Race, Ethnicity, and Health Disparities.” Ethnicity & Disease 29, no. 3: 513–16. https://doi.org/10.18865/ed.29.3.513.
Roblin, Douglas W., Thomas K. Houston II, Jeroan J. Allison, Peter J. Joski, and Edmund R. Becker. 2009. “Disparities in Use of a Personal Health Record in a Managed Care Organization.” Journal of the American Medical Informatics Association 16, no. 5: 683–89. https://doi.org/10.1197/jamia.M3169.
Romocki, LaHoma Smith, Andrea Des Marais, Leslie Cofie, Chelsea Anderson, Theresa Curington, and Jennifer Susan Smith. 2019. “Use of Short Messaging Service to Improve Follow-Up for Abnormal Pap Test Results in Minority and Medically Underserved Women in North Carolina: Questionnaire on Attitudes and Acceptability.” JMIR Formative Research 3, no. 3: e12675. https://doi.org/10.2196/12675.
Sarkar, Rahuldeb, Christopher Martin, Heather Mattie, Judy Wawira Gichoya, David J. Stone, and Leo Anthony Celi. 2021. “Performance of Intensive Care Unit Severity Scoring Systems across Different Ethnicities in the USA: A Retrospective Observational Study.” Lancet Digital Health 3, no. 4: e241–49. https://doi.org/10.1016/S2589-7500(21)00022-4.
Schmidt, Harald, Dorothy E. Roberts, and Nwamaka D. Eneanya. 2021. “Rationing, Racism and Justice: Advancing the Debate around ‘Colourblind’ COVID-19 Ventilator Allocation.” Journal of Medical Ethics, January. https://doi.org/10.1136/medethics-2020-106856.
Stevens, Nikki, and Os Keyes. 2021. “Seeing Infrastructure: Race, Facial Recognition and the Politics of Data.” Cultural Studies 35, no. 4–5: 833–53. https://doi.org/10.1080/09502386.2021.1895252.
Vega, Rosalynn Adeline. 2017. “Commodifying Indigeneity: How the Humanization of Birth Reinforces Racialized Inequality in Mexico.” Medical Anthropology Quarterly 31, no. 4: 499–518. https://doi.org/10.1111/maq.12343.
Vyas, Darshali A., Leo G. Eisenstein, and David S. Jones. 2020. “Hidden in Plain Sight—Reconsidering the Use of Race Correction in Clinical Algorithms.” New England Journal of Medicine 383: 874–82. https://doi.org/10.1056/NEJMms2004740.
Wang, Karen, Holly Grossetta Nardini, Lori Post, Todd Edwards, Marcella Nunez-Smith, and Cynthia Brandt. 2020. “Information Loss in Harmonizing Granular Race and Ethnicity Data: Descriptive Study of Standards.” Journal of Medical Internet Research 22, no. 7: e14591. https://doi.org/10.2196/14591.
Weber, Ellerie, Sarah J. Miller, Varuna Astha, Teresa Janevic, and Emma Benn. 2020. “Characteristics of Telehealth Users in NYC for COVID-Related Care during the Coronavirus Pandemic.” Journal of the American Medical Informatics Association 27, no. 12: 1949–54. https://doi.org/10.1093/jamia/ocaa216.
West, Suzanne L., William Johnson, Wendy Visscher, Marianne Kluckman, Yue Qin, and Ann Larsen. 2014. “The Challenges of Linking Health Insurer Claims with Electronic Medical Records.” Health Informatics Journal 20, no. 1: 22–34. https://doi.org/10.1177/1460458213476506.
Winner, Langdon. 1980. “Do Artifacts Have Politics?” Daedalus 109, no. 1: 121–36.
Young, Jennifer L., and Mildred K. Cho. 2021. “The Invisibility of Asian Americans in COVID-19 Data, Reporting, and Relief.” American Journal of Bioethics 21, no. 3: 100–2. https://doi.org/10.1080/15265161.2020.1870767.
Zhang, Xinzhi, Eliseo J. Pérez-Stable, Philip E. Bourne, Emmanuel Peprah, O. Kenrik Duru, Nancy Breen, David Berrigan, Fred Wood, James S. Jackson, David W. S. Wong, and Joshua Denny. 2017. “Big Data Science: Opportunities and Challenges to Address Minority Health and Health Disparities in the 21st Century.” Ethnicity and Disease 27, no. 2: 95–106.
Zhao, Yunpeng, Yi Guo, Xing He, Yonghui Wu, Xi Yang, Mattia Prosperi, Yanghua Jin, and Jiang Bian. 2020. “Assessing Mental Health Signals among Sexual and Gender Minorities Using Twitter Data.” Health Informatics Journal 26, no. 2: 765–86. https://doi.org/10.1177/1460458219839621.