On a More Comprehensive Governance of Artificial Intelligence
On a More Comprehensive Governance of Artificial Intelligence
In the last decade, advancements in artificial intelligence (AI) have found their way into various sectors, including predictive governance, healthcare, and generative media (e.g., ChatGPT and DALL-E). The increasing integration of AI technology into diverse areas has ignited a critical debate among policymakers, technologists, and scholars on responsible and ethical approaches to its deployment. The outcomes of this debate hold the key to shaping the regulatory landscape and the trajectory of future research and development in AI. However, amid the extensive discourse on AI governance, some of the most profoundly affected stakeholders and areas of action have been overlooked.
As national and international regulatory structures are being constructed, these have focused on the technical effects of AI, overlooking its production process and environmental impact. New governance mechanisms for AI address some of the technology’s potential harms but often overlook the relational, material, and political nature of data. Data, valuable in aggregation, is more about groups than individuals. It flows through physical infrastructures and relies on the labor of thousands of workers from countries like India, Kenya, and Venezuela. Furthermore, as an abstraction of reality shaped by decision-making, data is inherently political and never neutral.
As artificial intelligence continues to profoundly impact countless lives worldwide, it is crucial to ensure the effectiveness of this nascent legislation, given that governance has significantly lagged behind.
This essay is informed by my reading of the rapidly changing landscape of artificial intelligence governance, the growing scholarly body of work on the infrastructural and environmental impacts of AI, and my research into the outsourced labor that the technology industry employs. This labor is crucial for generating and annotating datasets and verifying algorithmic outputs for machine-learning techniques. My focus here is on recent AI legislation in Canada, the European Union, the United Kingdom, and the United States. As artificial intelligence continues to profoundly impact countless lives worldwide, it is crucial to ensure the effectiveness of this nascent legislation, given that governance has significantly lagged behind.
Data for Artificial Intelligence Depends on Human Labor
Over the course of my research, I have spoken with dozens of data workers from Latin America. The data-hungry AI industry procures some of it from outsourced workers ranging in age from children to the elderly and hailing from diverse regions, mostly the Global South. They contribute to data generation by taking photos of themselves, supply annotations for machine learning by providing labels for data points, and evaluate the accuracy of AI algorithms, to name a few examples.[1]Julian Posada, “Embedded Reproduction in Platform Data Work,” Information, Communication & Society 25, no. 6 (2022): 816–834. AI companies often exploit their labor to obtain data cheaply, taking advantage of international boundaries to circumvent labor regulations and access markets in which low wages and piecework prevail.[2]Paola Tubaro and Antonio A. Casilli, “Micro-work, Artificial Intelligence and the Automotive Industry,” Journal of Industrial and Business Economics 46 (2019): 333–345.
A prominent example of governance oversight neglecting data generated by human labor can be found in the Consumer Privacy Protection Act section of Canada’s Bill C-27, currently in committee, which focuses on data gathered through internet-based services. While privacy-related tools, such as informed consent and data erasure, are crucial, they should not be seen as the only solutions to data-production issues, because not all data derives from surveillance mechanisms. Data work, which forms a significant part of data production, intersects with labor rights, raising important questions on how to ensure that outsourced workers have decent wages, fair working conditions, and the right to organize. However, these areas are largely unaddressed by both national legislation and global AI governance mechanisms. This oversight exemplifies the limited, individualized view of data that fails to recognize that it is networked, distributed, outsourced, and intertwined with labor. In other words, data is not obtained exclusively from passive surveillance but also from active production processes embedded in larger systems of extractivism.
In the Canadian context, the Artificial Intelligence and Data Act, another component of Bill C-27, has the potential to address these issues. However, this hinges on the scope of the legislation’s definitions for harm—encompassing economic, physical, and mental impacts. These definitions are only effective if they are applied not only to the deployment of AI systems but also to their development, which means considering affected communities outside the country’s jurisdiction where the systems are deployed. While the country legislates within its borders, regulation should also consider the transnational nature of data flows and the responsibility of those importing the data. For instance, requiring global labor standards for data work, as exemplified by the Fairwork Project’s evaluations of working conditions in gig-economy platforms, is essential to mitigate human harm under that definition.
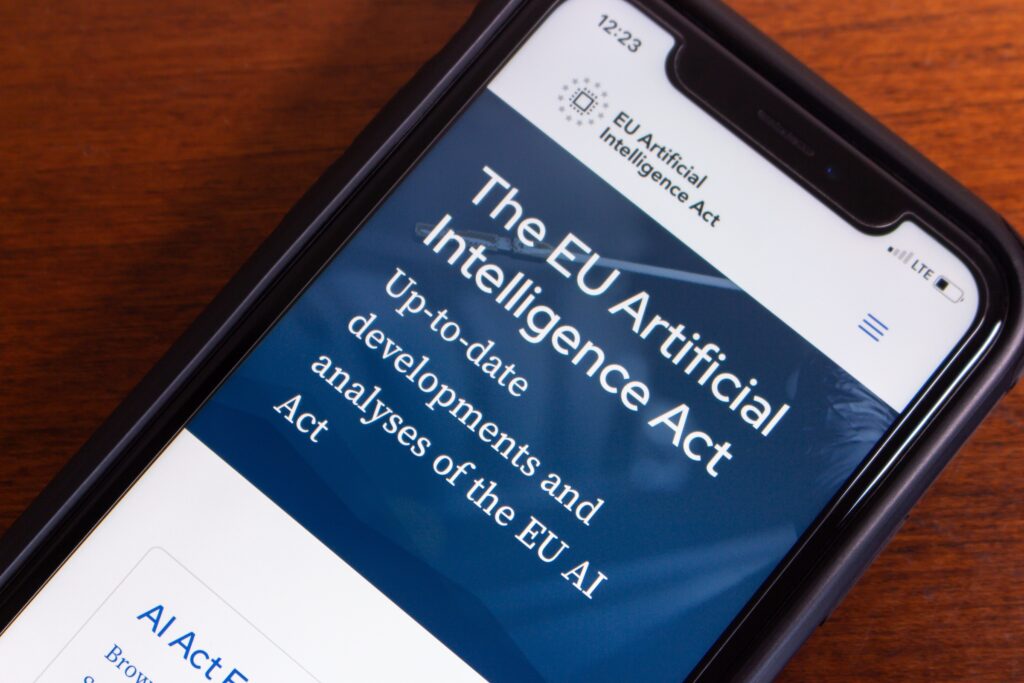
The Political Nature of Data Should Not Be Ignored
The focus on harms is a highlight of the EU’s recently approved Artificial Intelligence Act. This new piece of legislation categorizes AI in terms of risk, from unacceptable and high to minimal, with a special category for generative AI. Unlike other legislation, the AI Act slightly breaks with the consequentialist approach to AI regulation by auditing and assessing the quality of the data fed into the models, including its documentation and annotation processes, particularly for higher-risk systems, as well as focusing on the protection of fundamental rights, including labor rights.
Yet, both the EU’s AI Act and the UK government’s interim report on AI governance fall into the same pitfall by misjudging the politics of data and data-intensive systems like AI. Data, as an abstract representation of reality, is never neutral; it has inherent biases.[3]Catherine D’ignazio and Lauren F. Klein, Data Feminism (Cambridge, MA: The MIT Press, 2023). The interim report on AI governance considers machine-learning biases as risks rather than constant factors. Instead of focusing on deviations from neutrality, we should emphasize the importance of knowing whose perspectives shape the data used to train machine-learning models, what worldviews are encoded, and who is included, marginalized, or erased. As stated by information scholar Geoffrey Bowker, “raw data is an oxymoron,”[4]Geoffrey C. Bowker, Memory Practices in the Sciences, Inside Technology (Cambridge, MA: The MIT Press, 2006), 128. meaning that data is always “cooked”; it’s an abstraction of reality that carries specific meaning through collection and processing. By acknowledging the inherently political nature of data-driven AI—that it’s never neutral and always cooked—we can strive for governance mechanisms that drive progressive change and justice, rather than perpetuating inequalities.
Breaking with the ethos that any technology can be fixed and scaled up is a fundamental step in recognizing the inherent political nature of data and the limits of artificial intelligence in societal settings.
Due to the “impossibility of automating ambiguity,” in the words of computer scientist Abeba Birhane[5]Abeba Birhane, “The Impossibility of Automating Ambiguity,” Artificial Life 27, no. 1 (Winter 2021): 44–61.—essentially, the problems of quantifying, defining, and classifying humans—it is commendable that the prohibition of AI uses includes sectors such as public biometric identification, social scoring, and instances of human-behavior manipulation. Breaking with the ethos that any technology can be fixed and scaled up is a fundamental step in recognizing the inherent political nature of data and the limits of artificial intelligence in societal settings.
AI Governance Should Not Turn a Blind Eye on AI’s Environmental Impact
With the current paradigm of AI being bigger is better—meaning that more data and processing power are tied to more robust systems—companies are racing to increase their datasets, hardware, and processing centers. Recently, Nvidia became the most valuable public technology company thanks to its gamble on developing GPUs for AI. Google, which came to the fore a few years ago for firing members of its AI Ethics team over a paper critical of AI’s environmental impact,[6]Karen Hao, “We Read the Paper that Forced Timnit Gebru out of Google. Here’s What It Says,” MIT Technology Review, December 4, 2020. reported that its emissions have increased by 48 percent instead of meeting its net-zero goals.
AI governance should not ignore the environmental cost of technology. The recent Executive Order on the Safe, Secure, and Trustworthy Development and Use of Artificial Intelligence in the United States includes addressing AI’s environmental impact among its aims. Although most of the executive order focuses on national security risks, the section promoting innovation mentions the potential use of artificial intelligence to “streamline permitting and environmental reviews while improving environmental and social outcomes” and “mitigate climate change risks.” However, this consequentialist approach, once again, fails to address the impacts of AI development on the issue at hand.
This and other initiatives should not fail to consider the resources that underpin the technology. These resources include the electricity and water consumed by processing and data centers and the materials that make up the infrastructure sustaining AI, including minerals sourced worldwide.[7]Kate Crawford, Atlas of AI: Power, Politics, and the Planetary Costs of Artificial Intelligence (New Haven, CT: Yale University Press, 2022). For example, data centers in arid regions such as Arizona contribute to water scarcity, as well as the water used in the development of microchips, not to mention the potential disruption to communities inhabiting the territories where these infrastructures are built. The material and environmental aspects of AI raise fundamental questions that require addressing in our time.
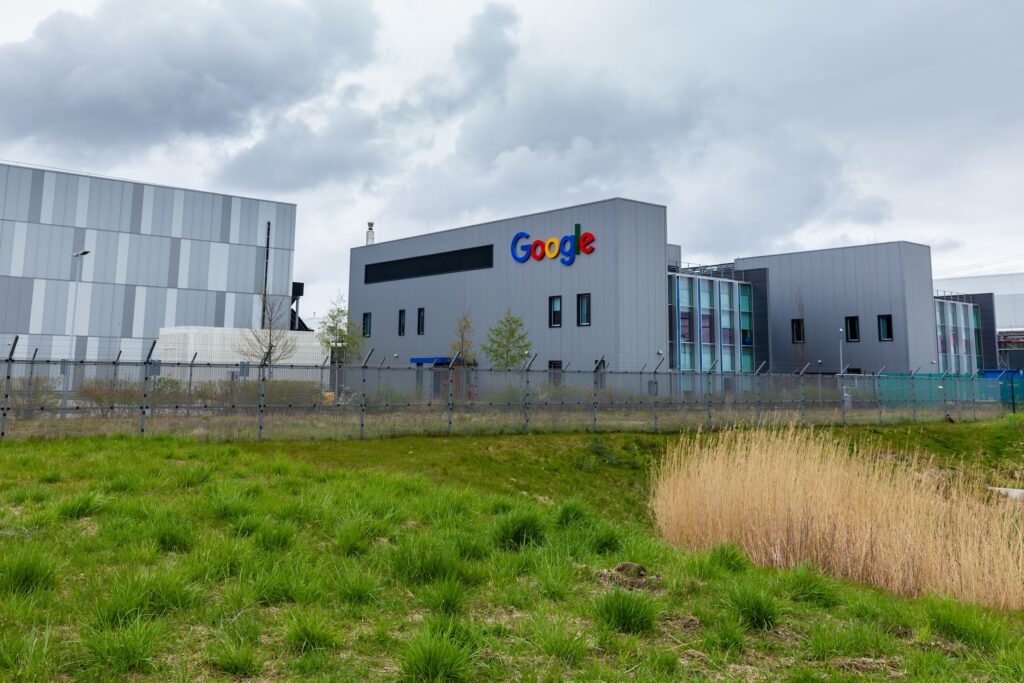
Prioritizing Human and Environmental Considerations in AI Governance
These examples shed light on the diverse dimensions of data, encompassing its relational, environmental, and political aspects. Consider the role of data workers as a prime illustration of how AI technology relies on the collective efforts of thousands, even millions, of individuals who curate and provide data. Their labor thrives in part due to the infrastructure of the internet, which not only enables online work but also underpins AI development. Paradoxically, when AI developers frame their work as a potential source of bias—suggesting that workers transmit their errors and opinions when working on the data—it leads data suppliers to reduce workers’ agency and increase control and surveillance. This process inadvertently allows the biases of their employers to permeate datasets and algorithms.
Addressing these pitfalls necessitates a paradigm shift in AI governance. We must recognize that data comes from interconnected individuals, making it inherently relational. Artificial intelligence is not ethereal but grounded in infrastructure reliant on natural resources, rendering it material. Data is never raw or neutral but inherently political, shaped by myriad perspectives and interests. Failing to recognize these characteristics of data and AI perpetuates pressing problems that disproportionately impact marginalized communities, including millions of data workers, the communities affected by resource extraction for AI infrastructure, and those individuals erased under a façade of neutrality. Artificial intelligence is not just about numbers and calculations; it is about people. Its impact transcends the boundaries of advanced economies, affecting communities worldwide. Embracing the multidimensional nature of AI and data is essential to ensure responsible and equitable AI governance for all.
The emerging governance landscape for artificial intelligence represents a pivotal advancement beyond the ethical debates often criticized for their lack of practical implementation. As we move forward, it is imperative that legislation and governance mechanisms prioritize the human and environmental dimensions of artificial intelligence, rather than focusing solely on its technical aspects. The future of this technology will not be determined by regulatory frameworks alone but by its tangible impacts on labor, environmental sustainability, and political equity. Ultimately, AI will be judged by its real-world consequences on those who work within its sphere, endure its environmental footprint, and navigate its political implications.
Footnotes
↑1 | Julian Posada, “Embedded Reproduction in Platform Data Work,” Information, Communication & Society 25, no. 6 (2022): 816–834. |
---|---|
↑2 | Paola Tubaro and Antonio A. Casilli, “Micro-work, Artificial Intelligence and the Automotive Industry,” Journal of Industrial and Business Economics 46 (2019): 333–345. |
↑3 | Catherine D’ignazio and Lauren F. Klein, Data Feminism (Cambridge, MA: The MIT Press, 2023). |
↑4 | Geoffrey C. Bowker, Memory Practices in the Sciences, Inside Technology (Cambridge, MA: The MIT Press, 2006), 128. |
↑5 | Abeba Birhane, “The Impossibility of Automating Ambiguity,” Artificial Life 27, no. 1 (Winter 2021): 44–61. |
↑6 | Karen Hao, “We Read the Paper that Forced Timnit Gebru out of Google. Here’s What It Says,” MIT Technology Review, December 4, 2020. |
↑7 | Kate Crawford, Atlas of AI: Power, Politics, and the Planetary Costs of Artificial Intelligence (New Haven, CT: Yale University Press, 2022). |