From Market Information to Management Information: Race and Data at the United States Employment Service
From Market Information to Management Information: Race and Data at the United States Employment Service
The potential uses (and abuses) of racial data lie at the heart of many controversies in contemporary US politics. One of the most high-profile incidents occurred in June 2023, when the US Supreme Court ruled in Students for Fair Admission v. Harvard against considering racial and ethnic information in college admissions. The logic behind the decision, a significant setback for efforts to diversify higher education, is that the most effective way for US institutions to not be racist is for them to simply ignore race—to be “colorblind.”
The notion that decisionmakers should not see “race” is not necessarily a new idea, having been repeated many times over the last several decades. Today, such colorblind rationales are increasingly being coded into the computerized systems used in the administrative state and other decision-making institutions. From the race-neutral algorithms increasingly used in the US court system to AI-powered hiring systems private employers use to screen job applications, colorblind computing is often framed as a technical solution to racial inequities. Technology studies scholars, however, have critiqued these and similar projects, arguing that these systems merely reify inequitable decision-making while giving it a veneer of computerized objectivity.[1]Ruha Benjamin, Race After Technology: Abolitionist Tools for the New Jim Code (New York: John Wiley & Sons, 2019).
Interrogating such systems from a historical perspective, we find that their putative race neutrality is really just their “frontstage” work. By paying attention to their “backstages”—in particular, to administrative conflicts over the kinds of data that should be produced and processed within government computers—we get a very different view of the sociotechnical systems that structure US racial order. My own research addresses this topic by investigating a seemingly mundane episode in the history of government computing: the computerization of the United States Employment Service (USES) beginning in the 1960s. In public-facing statements, USES leadership, charged with accusations that the agency discriminated against Black clients, described the computerization project as a means of instituting race-blind decision-making into their job-matching functions. Behind the government curtain, however, USES staff and management were engaged in a debate over the proper uses for racial and ethnic information within the agency. At stake in this conflict was less the issue of whether or not the USES should “see” race, but rather which particular people should have access to racialized data. In the case of the USES, racial data ultimately became a tool for agency managers, while disempowering frontline staff and clients.
The rest of this essay unpacks the controversy over colorblind computing in the USES. After contextualizing USES data practices in the following section, the subsequent sections trace three different conceptualizations of “racial data” that were invoked during the USES computerization project—racial data as a code, as an auditing technology, and as a tool of workplace control. I conclude by reflecting on how attending to these different conceptualizations can challenge scholars to engage with the shifting meanings of racial data throughout the design and use of computerized systems.
The USES, Data Cards, and the Datafication of Race
Created in 1933 by President Franklin Delano Roosevelt, the USES’s initial mandate was to provide job-finding services for unemployed Americans during the Great Depression (1929–1939). The USES established offices in cities around the country, which served as local labor exchanges that matched job seekers with open jobs in the region. Accomplishing this required USES staff to turn workers into data, collecting key information about their work history, skills, and other relevant experiences in order to form a “word picture” of the applicant for prospective employers. Equipped with this information, counselors used the cards to match workers with job orders for positions they deemed them to be good fits.
In researching the history of the datafication within the USES, one of the most intriguing shifts I have come across while sifting data cards has been the changes to their system of racial classifications. Comparing cards from the 1940s to those from the 1970s, one notices a progressive de-racialization of worker data. In figure 1, a mock-up card from 1945 includes “Peter Gorski’s” race (“W” for white) as well as his national origin (Russian).
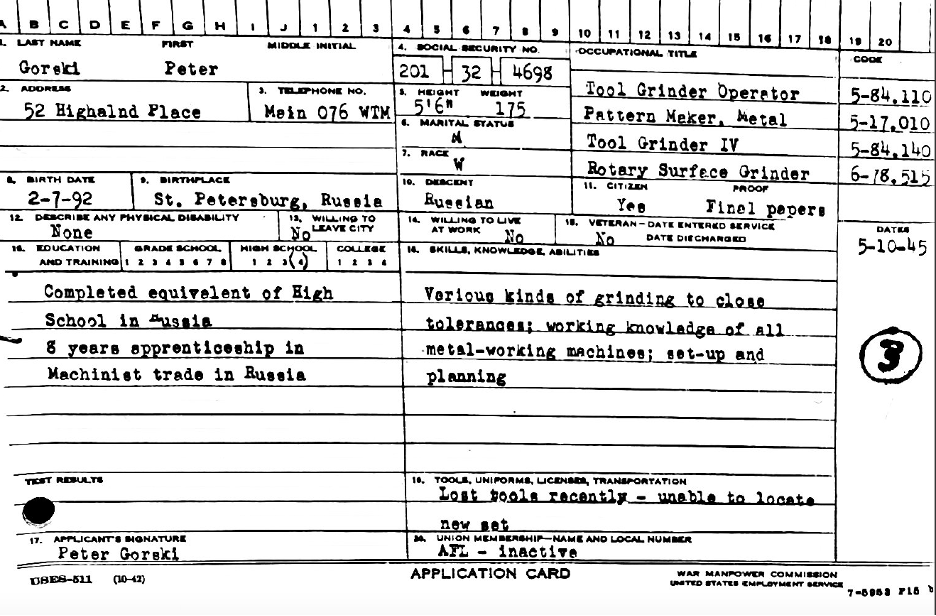
In 1968, the USES began pursuing the development of a nationwide computerized job-matching system, called the Job Service Matching System (JSMS). Even after computerization, the data card remained the central information technology for the agency’s operations. Computerized data cards, however, significantly differed from hand-written ones. Figure 2, a rendering of how worker data appeared on USES computer terminals in 1972, gives a sense of the new kinds of data categories that the JSMS collected, including psychological information pertaining to applicants’ aptitudes and interests. Most importantly, the computerized card contained no classifications for the applicant’s racial or ethnic background. In stark contrast to the handwritten cards of the 1940s, computerized data encouraged its viewers (typically employers and USES counselor) to imagine that it presented a word picture of anyone—and therefore of no one kind of person in particular. Technical manuals produced by the USES celebrated how the system’s “programming” prevented any “screening out or by-passing of a particular individual or job because of race.”[2]United States Employment and Training Administration (ETA), “Access to Automated Services for Rural Residents,” in Training for SESA [State Employment Security Agencies] Automation: A Developing … Continue reading
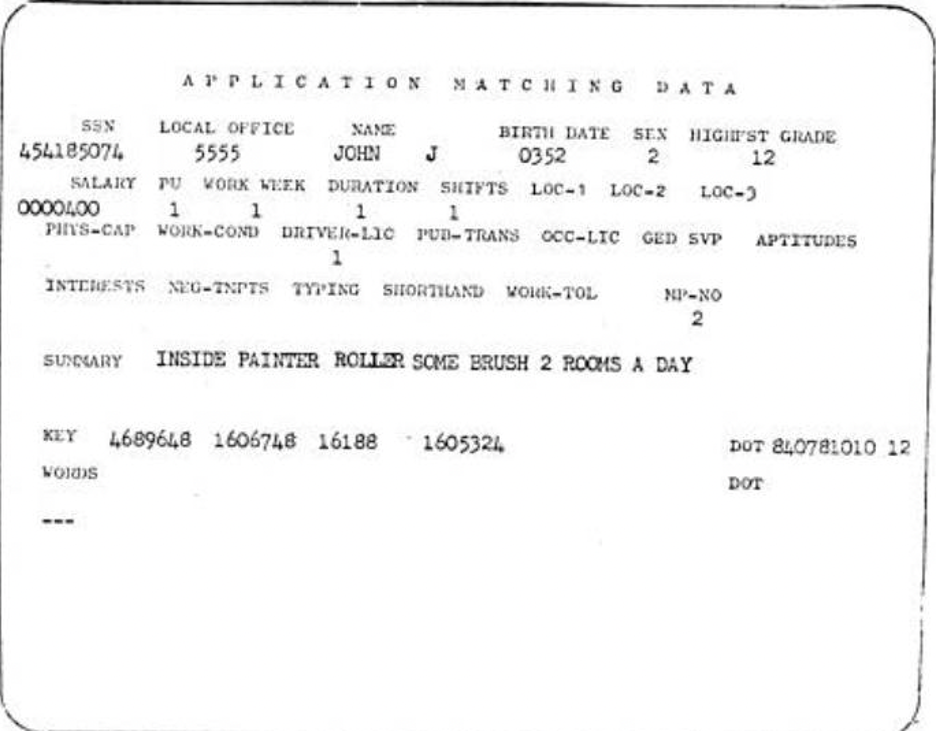
Why did this de-racialization of worker data proceed in step with the computerization of this very same information? Part of the answer lies within government discussions throughout the 1960s and 1970s about the potential discriminatory uses of racial and ethnic identifiers within government data systems. Within the USES, the dangers of racial coding were particularly acute, as civil rights leaders had long accused the agency of accommodating discriminatory job orders from employers that specified racial and religious hiring preferences, and of matching Black workers with jobs for which they were overqualified. These issues led the National Association for the Advancement of Colored People (NAACP) and other civil rights groups to vigorously oppose the coding of racial identification onto applicant data, due to its susceptibility for discriminatory uses. This campaign led to a federal ban on racial identification in the USES in 1961.[3]United States Commission on Civil Rights, Employment: 1961 Commission on Civil Rights Report, Book 3 (Washington, DC: US Government Printing Office, 1961), 116. However, subsequent investigations found that counselors continued to pencil in “x” marks onto cards or use other forms of covert coding to demark Black applicants’ data for discriminatory matching practices.
Computerization was intended prevent these kinds of practices, by taking job-matching out of the hands of counselors and assigning it to an automated computer program—what we today would call “an algorithm.” Rather than the older method of job-matching, which gave counselors the responsibility to choose which applicant was the best fit for an open job order, the JSMS automatically ranked work seekers based on how well their applicant profiles fit the required skills and experiences of a given job.
This system to render race imperceptible was the product of a set of ideas then emerging amongst economists and USES leaders that positioned race as a kind of code that structured both the work of the agency and the national labor market itself. By rendering race as code, they sought a computerized solution to the problem of employment discrimination.
Race as a Screening Device: The USES and Labor Market Information
As the USES developed the JSMS, agency leaders sought to situate computing as a technical solution to the problem of racial discrimination in the labor market. To aid in this effort, the service contracted economists from the Conservation of Human Resources Project at Columbia University to develop the conceptual foundations of a labor market information system that would inform their efforts to computerize the job-matching system.
The Conservation Project’s work for the USES built on research by labor economists that was reconceptualizing hiring discrimination as a statistical phenomenon tied to rational optimizing behavior pertaining to information costs and risks, rather than personal discriminatory tastes.[4]Representative examples of this work include Kenneth J. Arrow, “The Theory of Discrimination,” in Discrimination in Labor Markets, ed. Orley Ashenfelter and Albert Rees (Princeton, NJ: Princeton … Continue reading For these economists, discrimination occurred because “race” was an important signal that employers used to make sense of the imperfect information environment that constituted the labor market. In their report to USES leaders, Conservation Project researchers described the labor market as a “very complex but more or less automatic sorting device” in which employers tried to reduce costs via screening methods designed to eliminate individuals whose productivity they assessed to be low.[5]Boris Yavitz and Dean Morse, The Labor Market: An Information System (New York: Praeger Publishers, 1973), 16. According to this model, in the absence of individualized information about applicants, employers based their predictions of productivity on their own assessment of workers sharing the same social characteristics as them. The more information the employer has on the applicant as an individual, the more weight they place on that information; the less information they had, the more weight they placed on their perceptions of applicants’ social group. Thus, the absence of information led employers to discriminate.
By removing racial codes from the matching process, the JSMS was meant to resolve this issue by changing the information environment in which hiring took place and encouraging employers to see applicants as individuals rather than racialized statistics. Thus, when applicant data was retrieved from the central databank to identify a potential job match, there was putatively no possibility of discrimination against a particular individual or job because of social characteristics like race
Yet, despite the new system removing statistical discrimination from the job-matching process, race was—and still is—too deeply ingrained into US society for it to ever fully be automated away. One way of conceptualizing the absent-presence of race in the JSMS is through conceiving of it as a “ghost variable.” A “ghost variable” is a term that computer scientists use to describe an “entity in programming languages that do not correspond to any physical entities.”[6]Katrina Karkazis and Rebecca Jordan-Young, “Sensing Race as a Ghost Variable in Science, Technology, and Medicine,” Science, Technology, & Human Values 45, no. 5 (2020): 763–78, … Continue reading Science and technology studies (STS) researchers have more recently taken up the term to draw attention to how race continues to hold sway in technoscientific contexts in which it is submerged or disavowed.
One area in which we can discern the continued presence of racialized forms of knowledge in the USES job-matching system is in the kinds of psychological categories used to classify workers in the JSMS. This included a category of worker traits called the “negative temperaments” that were primarily used by counselors to code applicants with minimal employment histories, as an alternative to work experience or aptitude keywords. Whereas the listing of aptitudes indicated the potential range of jobs that a qualified applicant would thrive in, the negative temperaments indicated the kinds of work the hard-to-match jobseeker would likely find too difficult or overwhelming.[7]ETA, “Access to Automated Services for Rural Residents.” In trying to trace down the origins of the negative temperaments, I came across a 1971 study commissioned by the Office of Special Manpower Programs in the US Department of Labor (the executive agency that housed the USES) that purported to identify the common psychological characteristics of successful “escapees from the ghetto.” Titled A Study of Successful Persons from Seriously Disadvantaged Backgrounds, the study reviewed existing literature and administered a survey to 70 young Black and Mexican American young men who had been residents of “the ghetto section of some large city” to isolate the personal qualities that enabled some to become “successful”—in terms of educational and career achievement—while leaving others “unsuccessful” in their ability to find work or stay out of legal trouble. The goal of the study was to find a schema to differentiate “between the disadvantaged man who had been able to begin the pull upward and the disadvantaged man who did not or could not” to guide the efforts of the federal job matching and job training programs.
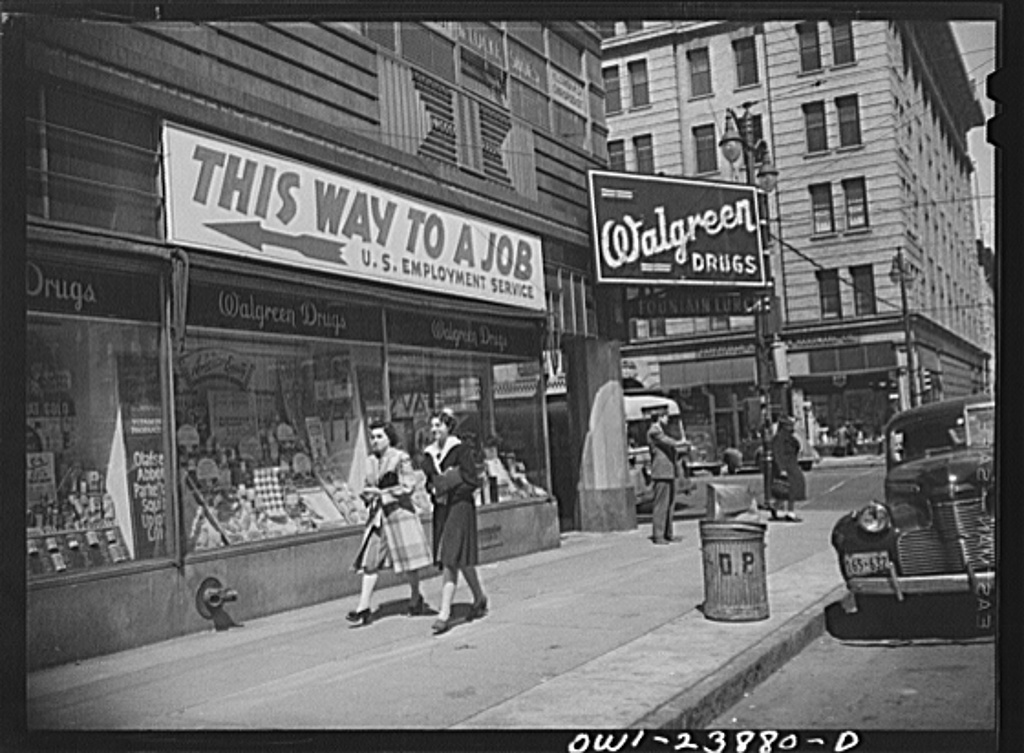
What is most interesting about the study is how the traits associated with the “successful” ghetto resident are the near inversed images of the negative temperaments that were standardized and coded into the JSMS. Whereas the “ghetto” study concluded that the successful ghetto-dweller engages in risk-taking behavior, one of the negative temperaments is that the job applicant did not perform well in emergency, critical, unusual, or dangerous situations. Where the successful ghetto-dweller was aware of alternative paths to accomplish goals, one of the negative temperaments was a dislike of variety or change in work duties and tasks. Successful individual: has supportive, inspiring relationships and a questioning orientation toward life and other people; negative temperament: cannot deal with people beyond giving and receiving instructions. Perhaps the most important distinction: while the successful individual possesses a sense of self, pride, and the feeling of being somebody, the difficult to match cannot convey any interpretation of their own feelings, ideas, or facts in terms of a personal viewpoint.[8]Edward M. Glazer and Harvey L. Ross, A Study of Successful Persons from Seriously Disadvantaged Backgrounds (Washington, DC: Office of Special Manpower Programs [USDOL], 1970).
While the JSMS’ race-blindness was premised on the notion that race was simply a kind of code that could be removed from applicant’s personal data, the way in which the negative temperaments echoed the characteristics of the unsuccessful ghetto-dweller suggest that “race” did not disappear from the computerized matching system so much as seep into new psychological conceptualizations pertaining to the purported cultural and moral failings of racialized people. The negative temperaments, which counselors assigned to applicants based on an initial intake interview, adjudicated between who was and who was not worthy of work in the labor market. They functioned as exclusionary codes in the JSMS’s matching program. If multiple applicants were found to be equally qualified for a position, those with the most negative temperaments would drop down the ranking order for the job match. While I was unable to find data on which types of individuals were most frequently coded with negative temperaments, investigations of the USES following computerization found that Black clients continued to be disproportionately referred to low-paying unskilled jobs throughout the 1970s.[9]US Government Accountability Office (GAO), Employment Service Needs to Emphasize Equal Opportunity in Job Referrals (Washington, DC: US Government Printing Office, 1980). In this way, “race” remained a far larger and more troubling presence than the efforts to remove racial classification from the JSMS would suggest.
The Hayward Experiment: Racial Data as an Auditing Tool
While the JSMS represented USES leadership’s desires to use new computer systems to make applicant information more readily accessible to employers, internal reformers of the agency thought that information technologies might be better put to use rectifying the informational imbalances of the labor market.
These ideas were put into practice in a USES office in Hayward, California, in the early 1970s. Many counselors and other staff at the Hayward office that joined the USES during that period had previously been part of labor unions and the civil right movement, and often shared similar social backgrounds to the job seekers they served. Seeing the failure of traditional USES methods to serve the needs of Black, immigrant, and poor clients, in 1970 they developed their own plan to overturn the informational order operating in traditional USES offices, as well as every “physical and procedural device that traditionally characterized the encounter between job seeker and bureaucracy.”[10]Miriam Johnson, Counter Point: The Changing Employment Service (Salt Lake City: Olympus Publishing Co., 1973), 124. “The Hayward Experiment,” as the counselors involved dubbed it, proposed an alternative informational order for the USES. Whereas in the traditional USES office, the “major activity is obtaining information from an applicant”; in Hayward, “the major activity is gathering and giving pertinent information to the applicant.”[11]Johnson, Counter Point, 129 (emphasis in original).
The experiment’s centerpiece was the occupational data bank, a series of printed files that collected every active job order in the area as well as ones the office had received in previous years. These were organized by occupation type in order to be easily retrievable and usable by applicants. By keeping older, inactive job orders available for USES clients to view, the Hayward office sought to allow different users to tailor the data bank for their own informational needs. This adaptability was most evident in the creation of specialized file groupings called “Ticklers.” These listed employers in relation to the information available on their hiring record for women, racial minorities, veterans, immigrants, and people with disabilities, as well as any available information on their positive or negative treatment of people from these social groups.
If the JSMS had sought to shape government data systems to “see” USES clients in ways amenable with the dictates of the job market, then the Hayward experiment sought to facilitate a much different goal: seeing like a marginalized citizen. More than a superficial tweak to established bureaucratic routine, the Hayward experiment challenged the informational asymmetries of Cold War-era bureaucracies in an effort to formulate an alternative model of how the state and its data systems could be brought to bear on the problem of racial and economic disadvantage.
ESARS and Racial Data as Workplace Control
The Hayward model gained popularity in the few years that it was active, with other offices across Northern California adopting the system. Nevertheless, the experiment ended when courts ruled in 1972 that these offices had to participate in the USES federally mandated data systems. This ruling resulted in the Hayward office having to take on both the JSMS and another automated data system, meant primarily to serve USES managers: the Employment Security Automated Reporting System (ESARS). ESARS was the official activity and service reporting system for USES. Every local office reported each job applicant registered, employer job order listed, and service provided to ESARS either directly through forms filled out by clerical staff or via interfaces with other data systems like the JSMS.[12]John M. Greco, Charles Fairchild, and Charles Fairchild, Determining the Management Information Needs of the United States Employment Service (Silver Spring, MD: Macro Systems, Inc., 1978). The system tallied these figures in monthly reports, which provided a quantified record of each offices’ performance.
Importantly, whereas race had been removed from JSMS data, it resurfaced within ESARS. The system provided information on the racial breakdown of clients served within USES offices, aggregated at the group level. Through this information, managers could evaluate the success—or failure—of their offices to place Black applicants into jobs. As managers pushed their offices to increase the placements of Black workers, racial data increasingly served as a means of workplace control.
ESARS was intended to promote a culture of managerial control and “accountability” at USES offices by strengthening managerial oversight over job placement. In so doing, it established the quantity of job placements—particularly for racial minorities—as the chief metric of success for USES offices. This new system was intended to help the most vulnerable; yet in practice, it often aggravated racial inequalities in USES services. The top-down injunction that “placements were the name of the game” led office managers to establish placement quotas and limit how much time counselors could spend with any one client.[13]GAO, Employment Service Needs to Emphasize Equal Opportunity, 18. As counselors in these offices complained, however, this often conflicted with their efforts to actually help improve the lives of the Black and poor clients they served. In a 1973 polemic on the current situation in the USES, one counselor who had worked in the Hayward office during and after ESARS’s experimental phase described how the “ESARS monstrosity” had led to a push to drive up placement numbers that often resulted in counselors sending applicants to lower-quality jobs.[14]Johnson, Counter Point. These jobs were the easiest to fill; they had the lowest level of employment qualifications, but they also paid worse and typically only offered temporary employment. Facing managerial pressures to find jobs for their clients, counselors found that they had no time to run personalized services like the “Ticklers” files, or even to provide additional guidance on skills like preparing resumes.
Instead, many counselors sought to artificially inflate their placement numbers by funneling their clients into the worst forms of work. Government investigations found that the incentive structures instituted through ESARS ultimately perpetuated racialized and gendered labor market inequalities.[15]GAO, Effect of the Department of Labor’s Resource Allocation Formula on Efforts to Place Food Stamp Recipients in Jobs (Washington, DC: US Government Accountability Office, 1979). The ultimate result was to trap Black applicants into an endless cycle of dead-end jobs, while providing managers with a sense of success.
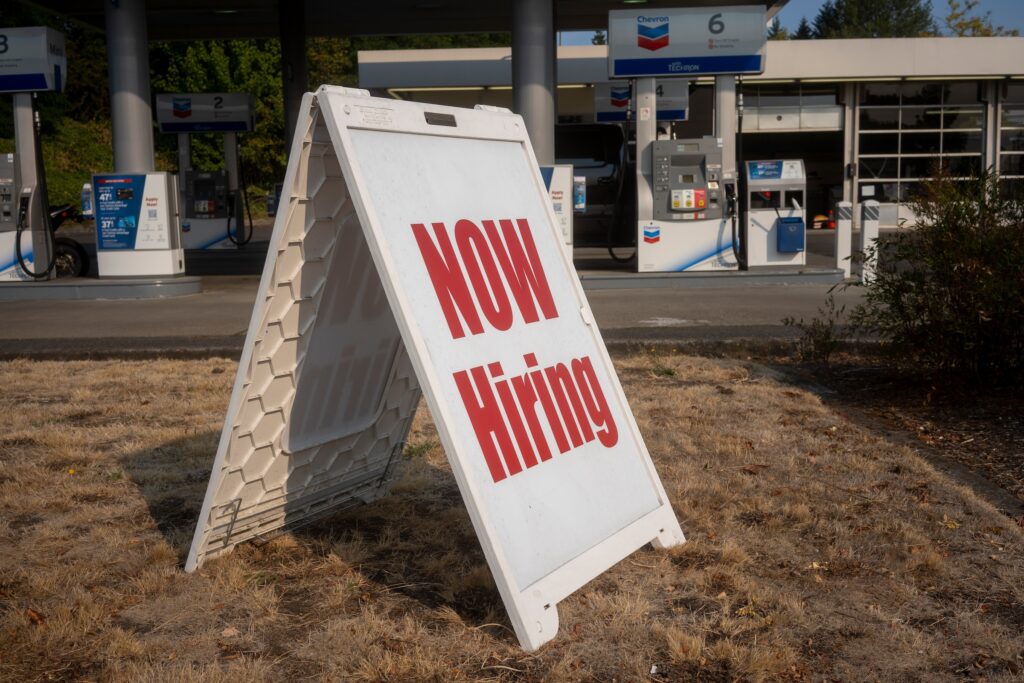
Conclusion
ESARS ultimately spelled the end of efforts to create alternative data systems that responded to the informational needs of marginalized citizens, as had been attempted in the Hayward experiment, and instead fully rendered racial data as a tool for facilitating market rationales and the prerogatives of USES managers.
USES leadership’s claims that computerization would “program” race out of the job-matching process was undercut by the persistent reality of the unequal treatment of racialized work seekers in USES offices. While both the JSMS and ESARS were intended to aid Black USES clients, in practice, they often channeled them into temporary, low-paying, and insecure jobs. While explicit racial codes had been removed from worker data in the JSMS, psychological categories like the negative temperaments ensured that racialized modes of differentiating those deemed worthy of work and those not were frequently used to make employment decisions. In effect, such proxy categories ensured that, for those who were trained to read between the lines of data, information about workers’ race was both easy to see and frequently used to make job-matching decisions. While ESARS nominally provided oversight over these forms of discrimination in the matching process, it instead further incentivized counselors to place Black applicants into the worst forms of work. Both systems also disempowered the efforts of USES frontline staff and their clients to shape data systems that might better serve the most marginalized jobseekers.
How might this history inform present-day conversations around color-blind computing and other modes of putatively race-neutral forms of decision-making? The most pertinent point is that critical analysis of these controversies must extend beyond the question of whether institutional systems should be “race conscious” or not. Instead, analyses need to trace the political struggles that shape the sociotechnical order of the information technologies of the state. This amounts to critically theorizing what STS scholar Michelle Murphy calls the “regimes of perceptibility” instituted through government data. Should government data systems facilitate “seeing like a state?” Seeing like a market? Or seeing like a marginalized citizen?
By asking such questions, scholars can also recover alternative imaginaries like the Hayward Experiment and other projects that were swept aside by struggles for bureaucratic control. Drawing attention to these roads not traveled is especially pertinent today as government officials, Big Tech, and Big Data boosters propose colorblind computing as a solution to a whole host of race-related social problems—from purportedly nondiscriminatory hiring algorithms to police data systems that automatically redact racial and ethnic information. What the history of USES computing underscores is that far from being “race blind,” such systems reveal race in very particular ways and to very particular people, often creating bureaucratic dynamics that tend to harm the very people “deracialization” is imagined to be aiding.[16]Alex Chohlas-Wood et al., “Blind Justice: Algorithmically Masking Race in Charging Decisions,” in AIES ’21: Proceedings of the 2021 AAAI/ACM Conference on AI, Ethics, and Society (New York: … Continue reading Nonetheless, there always exist options for alternative designs. The choice of which priorities and objectives we attach to technologies of governance is ours to make.
Footnotes
↑1 | Ruha Benjamin, Race After Technology: Abolitionist Tools for the New Jim Code (New York: John Wiley & Sons, 2019). |
---|---|
↑2 | United States Employment and Training Administration (ETA), “Access to Automated Services for Rural Residents,” in Training for SESA [State Employment Security Agencies] Automation: A Developing National Program for the State, Job Service, Unemployment Insurance Service (Washington, DC: Mark Battle Associates, Inc., 1976), 31. |
↑3 | United States Commission on Civil Rights, Employment: 1961 Commission on Civil Rights Report, Book 3 (Washington, DC: US Government Printing Office, 1961), 116. |
↑4 | Representative examples of this work include Kenneth J. Arrow, “The Theory of Discrimination,” in Discrimination in Labor Markets, ed. Orley Ashenfelter and Albert Rees (Princeton, NJ: Princeton University Press, 1974), 3–33; Albert Rees, “Information Networks in Labor Markets,” American Economic Review 56, no. 1/2 (1966): 559–66, https://www.jstor.org/stable/1821319. |
↑5 | Boris Yavitz and Dean Morse, The Labor Market: An Information System (New York: Praeger Publishers, 1973), 16. |
↑6 | Katrina Karkazis and Rebecca Jordan-Young, “Sensing Race as a Ghost Variable in Science, Technology, and Medicine,” Science, Technology, & Human Values 45, no. 5 (2020): 763–78, https://doi.org/10.1177/0162243920939306. |
↑7 | ETA, “Access to Automated Services for Rural Residents.” |
↑8 | Edward M. Glazer and Harvey L. Ross, A Study of Successful Persons from Seriously Disadvantaged Backgrounds (Washington, DC: Office of Special Manpower Programs [USDOL], 1970). |
↑9 | US Government Accountability Office (GAO), Employment Service Needs to Emphasize Equal Opportunity in Job Referrals (Washington, DC: US Government Printing Office, 1980). |
↑10 | Miriam Johnson, Counter Point: The Changing Employment Service (Salt Lake City: Olympus Publishing Co., 1973), 124. |
↑11 | Johnson, Counter Point, 129 (emphasis in original). |
↑12 | John M. Greco, Charles Fairchild, and Charles Fairchild, Determining the Management Information Needs of the United States Employment Service (Silver Spring, MD: Macro Systems, Inc., 1978). |
↑13 | GAO, Employment Service Needs to Emphasize Equal Opportunity, 18. |
↑14 | Johnson, Counter Point. |
↑15 | GAO, Effect of the Department of Labor’s Resource Allocation Formula on Efforts to Place Food Stamp Recipients in Jobs (Washington, DC: US Government Accountability Office, 1979). |
↑16 | Alex Chohlas-Wood et al., “Blind Justice: Algorithmically Masking Race in Charging Decisions,” in AIES ’21: Proceedings of the 2021 AAAI/ACM Conference on AI, Ethics, and Society (New York: Association for Computing Machinery, 2021), 35–45, https://doi.org/10.1145/3461702.3462524; Manish Raghavan and Solon Barocas, Challenges for Mitigating Bias in Algorithmic Hiring (Brookings Institution, 2019), https://www.brookings.edu/articles/challenges-for-mitigating-bias-in-algorithmic-hiring/. |